Conversion Rate Optimization (CRO) – A Complete Step by Step Guide
What is conversion rate optimization?
Conversion rate optimization (CRO) improves a website’s effectiveness by increasing the percentage of visitors who take a desired action, such as purchasing or signing up. Successful CRO uses methods such as A/B testing, behavioral analysis, and user experience improvements to maximize conversion rates.
What is conversion rate optimization useful for?
Online traffic can be unpredictable, if visitors don’t enter your conversion funnel right away, they’re less likely to return and take action. Thus, to improve your chances of increasing conversions, you need to run effective CRO campaigns.
A CRO campaign involves analyzing your current website experience, running tests, and personalizing experiences. If the changes positively impact KPIs, you make them permanent.
A solid CRO campaign saves you time, money, and effort while revealing new growth opportunities. It helps you understand your website’s usability, gain insights into customer behavior, and improve your UX to meet your goals.
Download the ‘Building a CRO-First Culture’ whitepaper to discover a clear and actionable framework for implementing CRO.
6 Important website elements for conversion rate optimization
In this section, we’ll explore how strategic design elements and well-crafted content can enhance user experience, increase engagement, and improve conversions, turning visitors into loyal customers.
1. Landing page design
Landing page design is crucial for a website’s usability and success. A well-designed site attracts more visitors.
Take Amazon’s product pages as an example. Most customers visit with the intent to purchase. Amazon’s design maximizes conversions by making key elements easy to find. For instance, the “Add to Cart” button is prominently displayed in bright orange next to the product details. This color stands out against the white background, drawing attention and prompting action.
Additionally, Amazon uses white space effectively to highlight product features and large images to the left, building trust and quickly engaging website visitors.
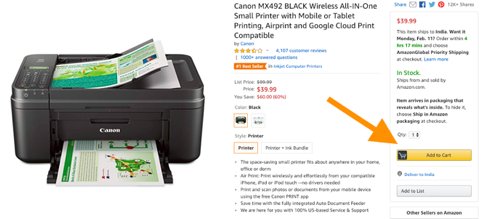
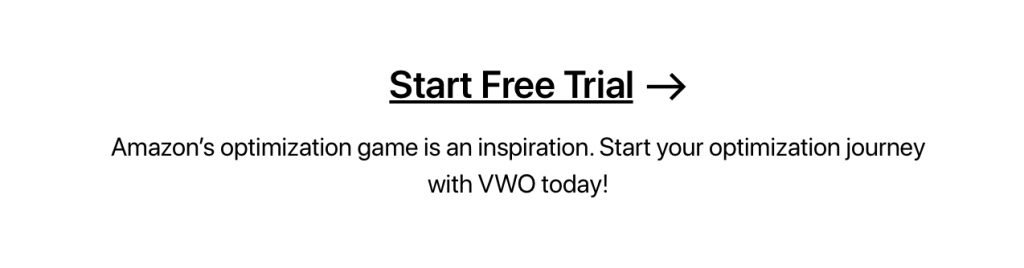
2. Website copy
Website copy plays a vital role in converting website visitors into leads. While a well-designed site can attract traffic, engaging content persuades website visitors to take action or leave without doing anything. Effective website copy consists of two key elements:
- Headline
Your headline is the first thing website visitors see. It shapes their first impression and determines whether they stay. To make it effective:
- Formatting: Choose a readable font, size, and color to grab attention.
- Writing style:
- Ask a question: e.g., “Did you know email marketing can increase your revenue by 30%?”
- Split content into parts: e.g., “Internet Marketing: What’s in store?”
- Address directly: e.g., “Can you rely on content marketing?”
- Focus on numbers: e.g., “10 ways to boost your conversions with email marketing.” Keep it concise and clear, focusing on what your product or service offers.
- Ask a question: e.g., “Did you know email marketing can increase your revenue by 30%?”
- Formatting: Choose a readable font, size, and color to grab attention.
- Body content
Your body content answers, “What’s in it for me?” It should be clear, concise, and represent your brand well. To craft compelling body content:
- Formatting:
- Break text into short paragraphs for easy reading.
- Use subheadings to organize information.
- Include bullet points or numbered lists when needed.
- Break text into short paragraphs for easy reading.
- Writing style:
- Match the tone to your target audience (fun, professional, casual).
- Use stylistic elements like metaphors to emphasize key points.
- Directly address the user’s needs and questions.
- Include key phrases for better usability and clarity.
- Match the tone to your target audience (fun, professional, casual).
- Formatting:
For example, Slack uses a strong headline followed by clear content that highlights its unique selling points, making it easy for website visitors to understand its value.
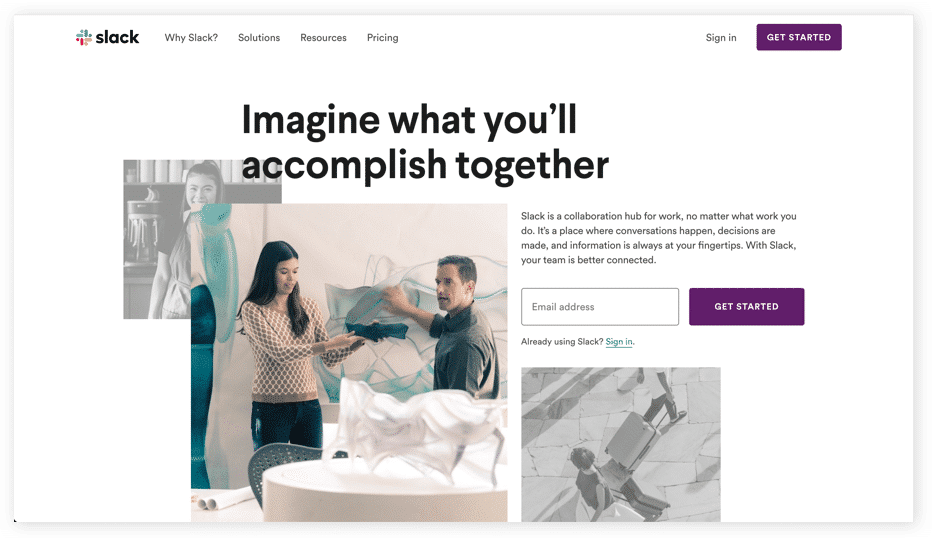
A catchy headline accompanied by content that’s concise and answers all the necessary questions makes any page look attractive and does the desired work – getting customers onboard.
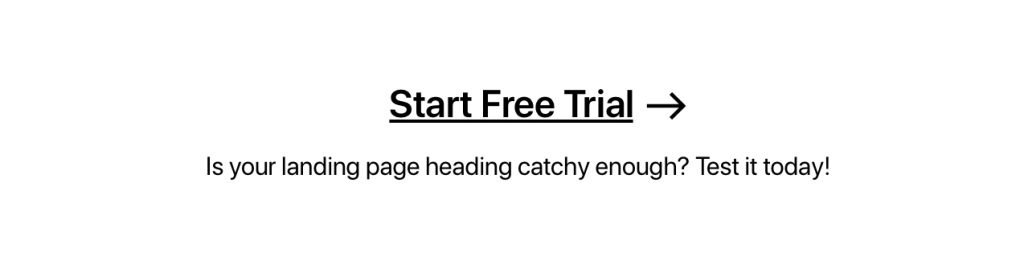
3. Call-to-action
A call-to-action (CTA) asks customers to take a specific action, like subscribing to a newsletter, booking a webinar, or making a purchase. The clearer and more compelling your CTA, the more leads it generates.
Industry leaders use basic psychology to shape their CTAs. For example, ADT increased conversions by 60% just by changing the text of their CTA from “Book a Free Survey” to “Get a Free Quote.”
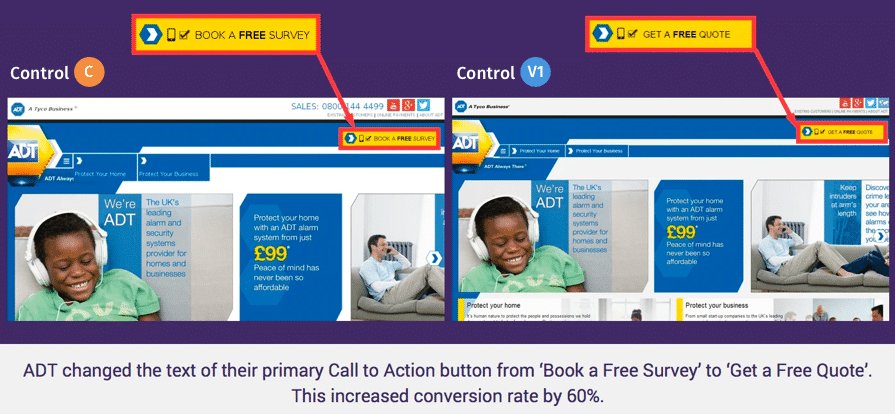
4. Navigation and site structure
Your site’s structure should focus on creating an easy-to-navigate experience. At its core, site structure is a map of how your pages interact with each other.
While every site is unique, the basic navigation hierarchy is similar. You start from the homepage, then explore categories and subcategories until you find what you’re looking for. If this process is smooth, users won’t have trouble navigating. But if it’s unstructured, they’ll get lost and leave.
To ensure easy navigation, make sure users can move quickly between important sections and find what they need with as few clicks as possible. A well-organized site boosts conversions and enhances your brand reputation.
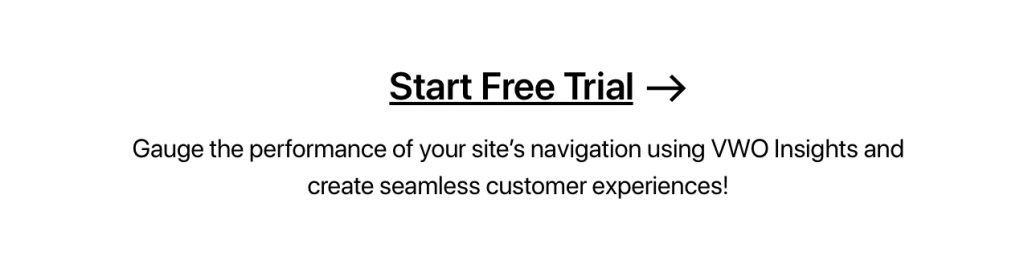
5. Forms
Forms are key to most businesses, especially in the sales funnel. Optimizing them can significantly boost conversions. While there are many theories about creating effective forms, what works depends on your business. Sometimes, a comprehensive form is needed, while in other cases, a simple form works better. The key is balancing lead quality and volume for the best ROI.
Here are three tips to optimize your forms:
- User-friendly design: Clear, consistent styling, tooltips, and validation improve user experience. Prioritize important fields and experiment with progressive forms.
- Simplified password creation: Help users create easy-to-remember passwords to speed up the form-filling process.
- One-click submissions: Allowing one-click sign-ups via Facebook or Google makes conversions easier, especially if users are already logged in. However, this might not work for all businesses, especially B2B.
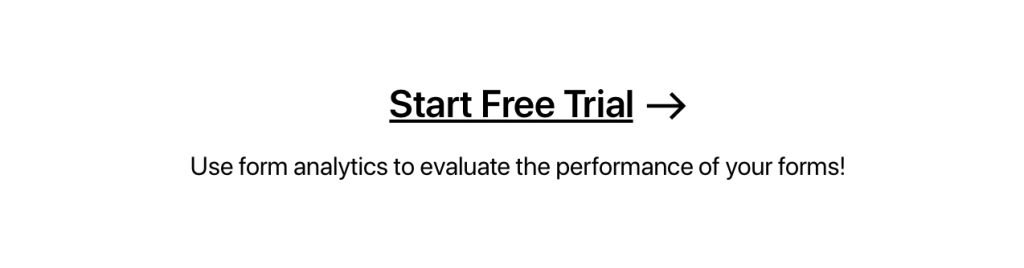
6. Page speed
Page speed directly impacts your site’s performance, user experience, conversion rates, and search engine ranking. According to Semrush, if your site loads in 1.7 seconds, it’s faster than 75% of the web. At 0.8 seconds, it beats 94% of sites.A one-second delay can reduce conversions by 7%. For example, if your site earns $100,000 a day, that delay could cost you $7,000 or more daily.
“If you click a page and you see some credit card fields that you weren’t expecting to see, you will automatically exit that page. Combat this by letting users know they will be redirected to a checkout page.” – Vlad Bisceanu, Marketing Director at UltiMaker
Source: VWO Webinar
5 Conversion rate optimization steps: Understanding the process
At its simplest, the conversion rate optimization process can be divided into 5 steps.
Step 1: The research phase – Identifying the areas of improvement
Many marketers often replicate successful CRO processes and strategies from other businesses. But it’s no one-size-fits-all approach.
To understand user actions, start by analyzing quantitative data like bounce rates, traffic sources, demographics, and more on your website. Next, for qualitative analysis, analyze session recordings, heatmaps, and surveys to understand the “why” behind visitors’ actions.
By combining quantitative and qualitative data, you have a comprehensive understanding of visitor behavior. This helps you make strategic, tailored improvements that benefit your business in the long run.
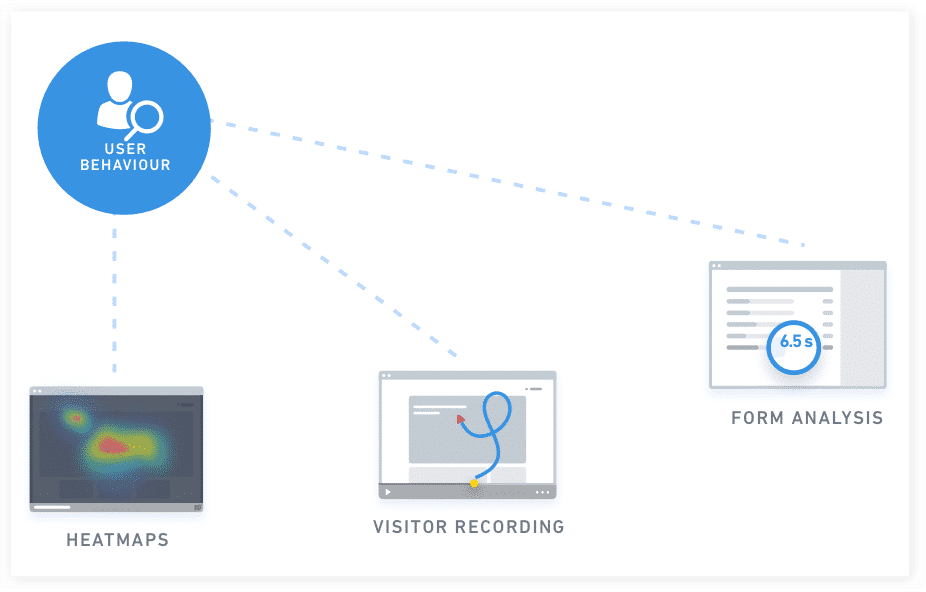
Step 2: The hypothesis phase – construct an educated hypothesis
Remember, a logical hypothesis has three parts:
- A specific change based on behavioral research
- A desired effect (a conversion metric, for example)
- The reasoning behind the change.
For instance, “Adding social proofs on product pages will result in a 5% increase in add-to-carts because visitors are more likely to feel confident in purchasing if they see validations.” A well-structured hypothesis guides optimization efforts and keeps your testing program in the right direction.
Step 3: The prioritization phase – choose an order
Here, a number of frameworks can help you through the process. Of these, the P.I.E. framework formulated by Chris Goward at WiderFunnel is what we most recommend:
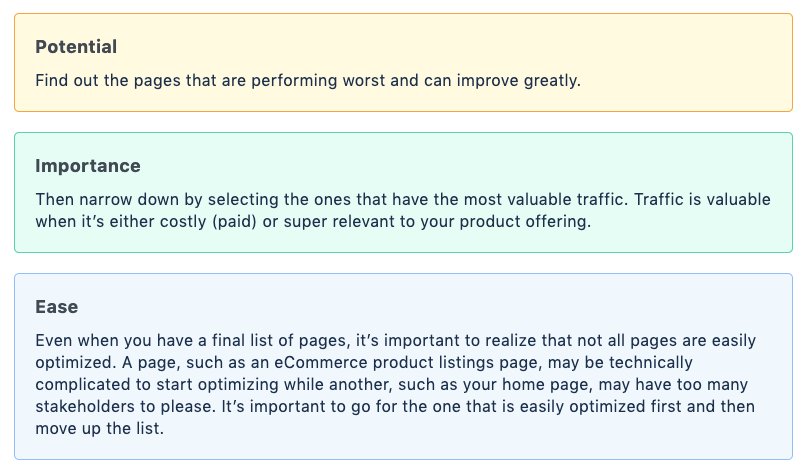
Each of these has its own importance attached which can effectively help in prioritizing your testing elements and take you in the right direction.
Step 4: The testing phase — A/B, split, or multivariate?
Before running a test, understand the basics:
- What is statistical significance, and why is it critical?
- How long do you need to run a test?
- What should I use—A/B, Split, or Multivariate test?
A significant result means you can trust that the changes you see are real, not just luck. For example, if a test shows a 93% statistical significance, there’s only a 7% chance it happened by accident. This suggests it’s the right time to end the test, provided it has run long enough for reliable conclusions. Use our testing duration calculator to decide the statistical significance of your test.
Next, you decide which type of test you want to run: A/B testing, Split testing, Multivariate testing.
Note: Download the Experimentation Program Maturity Report 2024 to explore strategies from 206 companies and learn key insights for driving effective experimentation.
Step 5: The learning phase – how to analyze A/B test results
This phase is where you analyze your test results, complete the testing loop, and gather insights for future tests. Don’t be done with checking if a variation won or failed. It’s important to go deeper in your analysis. If you have a winner, check if the costs of changes are justified by expected revenue. In case of a loss, see it as a learning opportunity and delve into your research, break down the data, find insights, and refine your hypothesis for future tests.
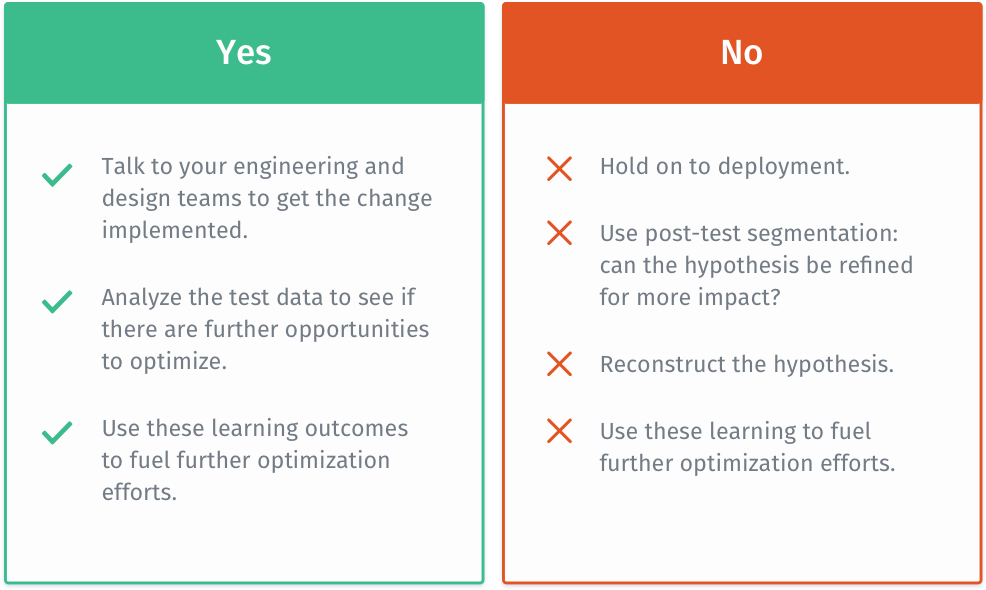
“It’s very important that whatever challenges you face, whatever operational learnings you have, you document. And lastly, sharing and evangelizing the learnings because you can have real success from conversion rate optimization efforts when you are sharing the learnings with other teams.” – Nancy Thakur, Head of CRO at Mindvalley
Source: VWO Webinar
Industry-specific conversion rate optimization
Conversion rate optimization is beneficial for businesses of all types and sizes, irrespective of their industries. Here are some interesting business use cases defining the pervasive nature of conversion rate optimization.
CRO for B2B/SaaS companies
Lead generation is the initiation of grabbing the interest of your customers by engaging them on your site, gathering their information through various means (forms, sign-ups, surveys, etc.), and contacting them to convert them into loyal, recurring customers. As a B2B/SaaS business, it becomes your duty to help your customers find what they’re looking for, capture their interest, and support their buying decisions.
Case study: Continuous testing helped POSist improve their demo requests by 52%!
A continuous-testing program on POSist’s homepage and contact page added laser-focused copy around value drivers (mention example changes here) and tightened the demo form. The result: +52 % demo requests and a 3.4 % site-wide conversion rate – a 25 % lift.
Read the full success story of POSist.
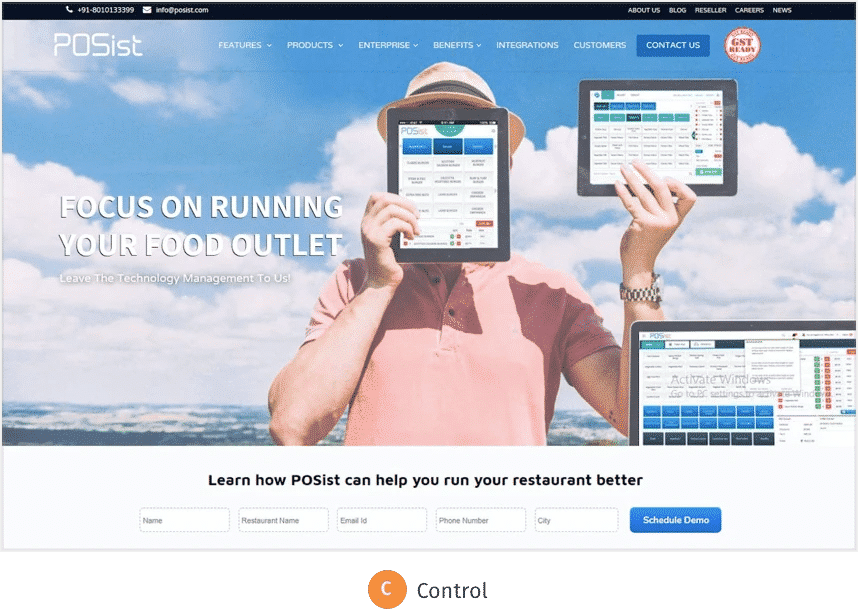
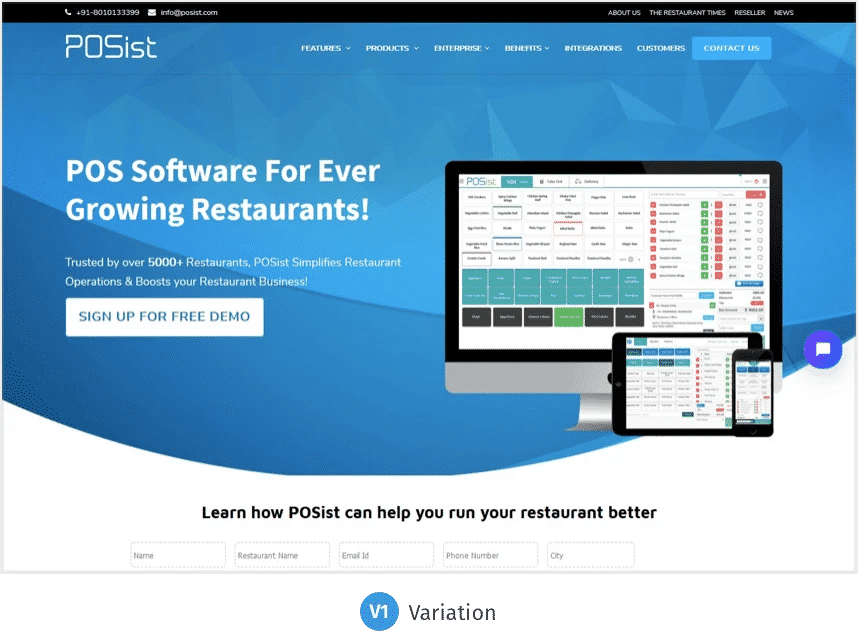
CRO for an eCommerce business
Shopping cart abandonment is a major challenge for eCommerce companies, costing billions in lost revenue each year. According to the Baymard Institute, 69% of eCommerce visitors abandon their carts for various reasons. To reduce abandonment and improve eCommerce conversion, create a user-friendly site with great design, exclusive products, and clear shipping costs. Also, address issues like complex payment processes and missing information.
Common reasons for cart abandonment include:
- Distractions: Too many pop-ups or forms.
- Difficult checkout: Complicated checkout for first-time users.
- Hidden costs: Unexpected charges like shipping and taxes.
Running a CRO campaign helps identify and fix these issues, improving conversions.
Case study: PearlsOnly increased its revenue by 12% by optimizing its checkout page!
PearlsOnly, an online jewelry store in Houston, specializes in selling pearls. They found that a cluttered checkout page caused visitors to abandon it. With VWO’s help, PearlsOnly optimized the checkout page and highlighted key selling points, which helped them see a 10% increase in revenue.
Read the full success story of PearlsOnly.
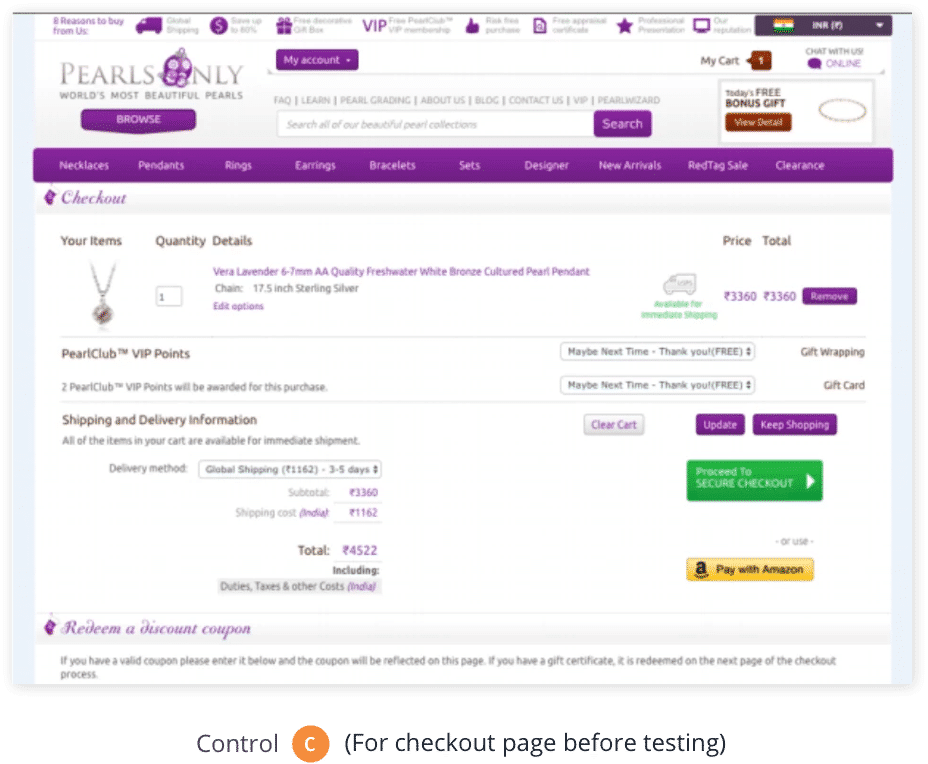
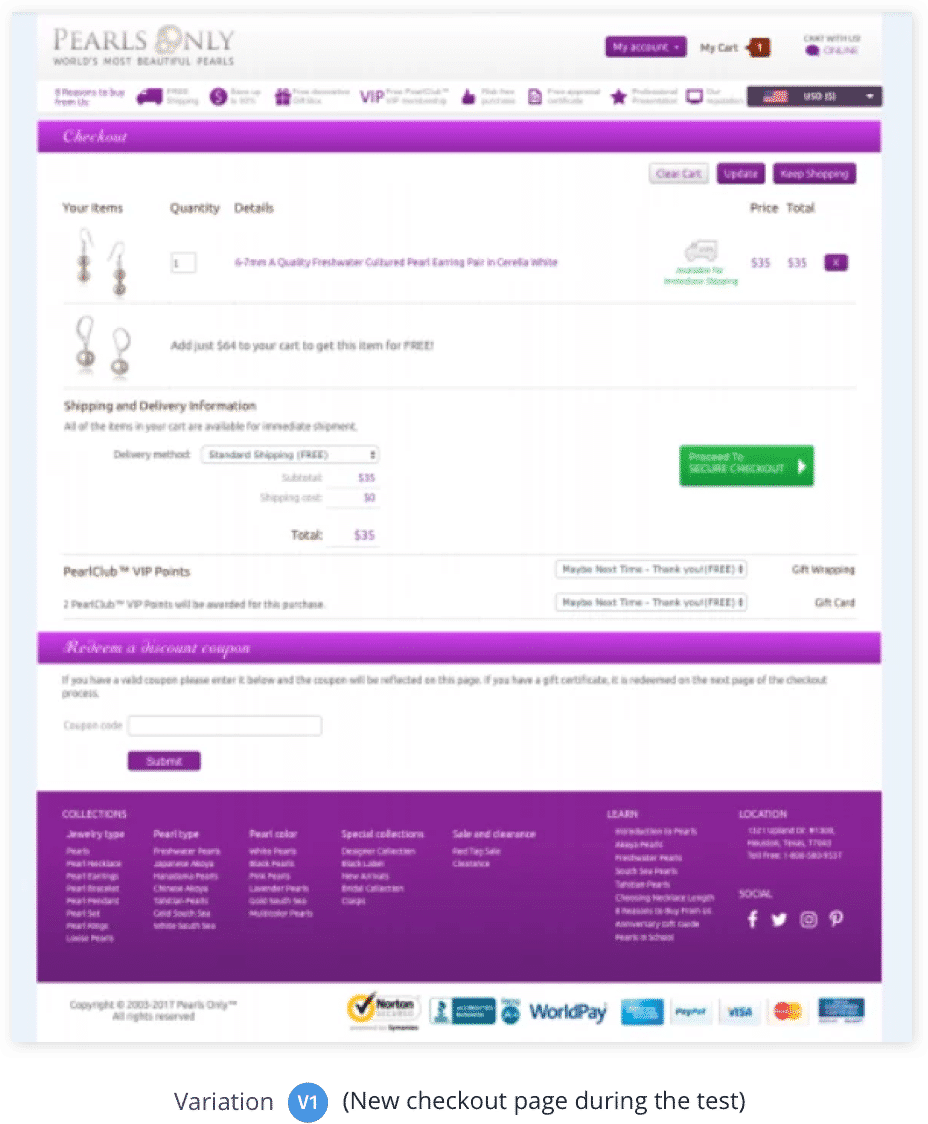
CRO for Media/Publishing houses
For businesses like media agencies and publishing houses, reaching a larger audience base and keeping them engaged on their platform is crucial for their growth. Here, the CRO can help them with this and test various site elements such as email sign-ups, social sharing icons, recommended content, and other promotional options to capture more attention.
Case Study: BluTV increased its mobile conversion rate by 42% by testing the homepage!
BluTV, an OTT service, aimed to convert visitors into paid trial subscribers. VWO partner Hype identified low mobile conversions and revamped the homepage by removing distractions and adding an FAQ section. A 19-day split test resulted in a 42% increase in conversions.
Read the full success story of BluTV.
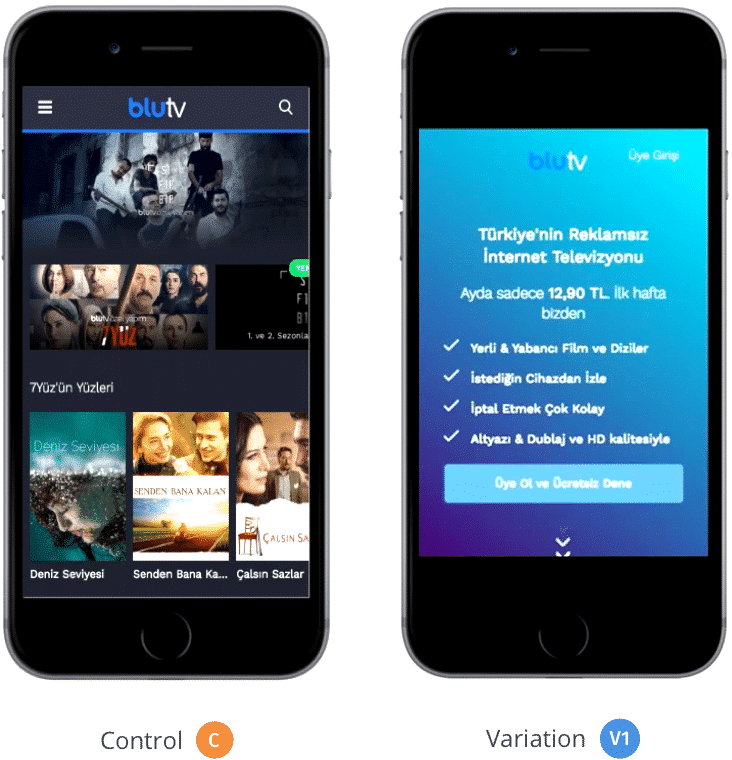
CRO for OTAs or Travel agencies
Compared to other industries, travel companies usually face greater difficulties in getting conversions. Consumers in the category tend to take longer than usual to decide whether or not to make a purchase. They browse through various sites, compare deals, interact with their peers, and only then take a call. Further, complex booking behavior also adds to the industry’s challenge. Running a CRO campaign can effectively help travel companies analyze their potential and drawbacks and enhance conversions.
Case study: Bizztravel Wintersport increased its conversions by 21%!
Bizztravel Wintersport is a Dutch travel company that improved its conversions by 21%, simplifying how users searched for holiday destinations on their website. The company decluttered its site’s homepage by keeping only the most relevant information intact.
Read the full success story of Bizztravel Wintersport.
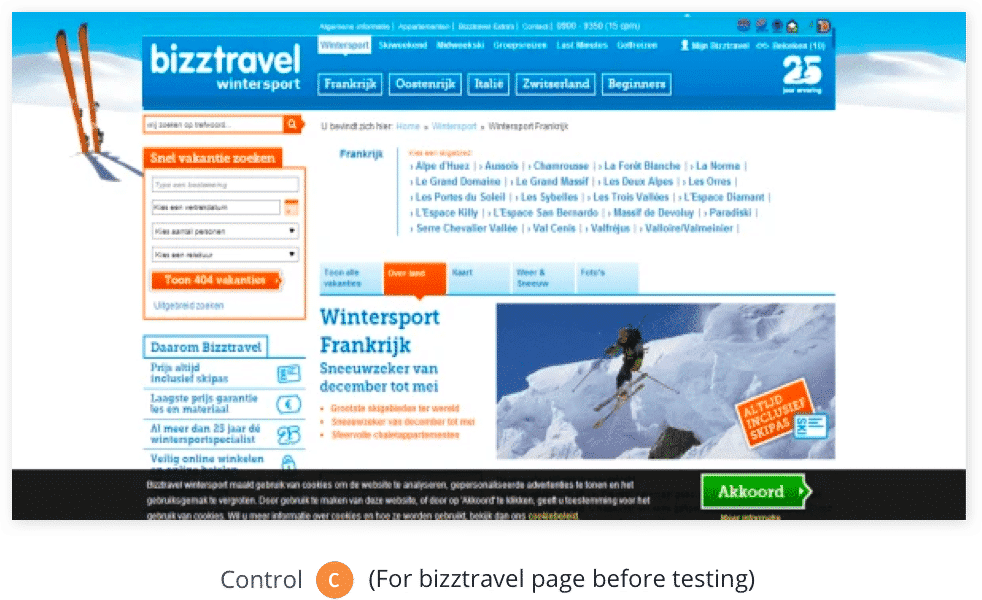
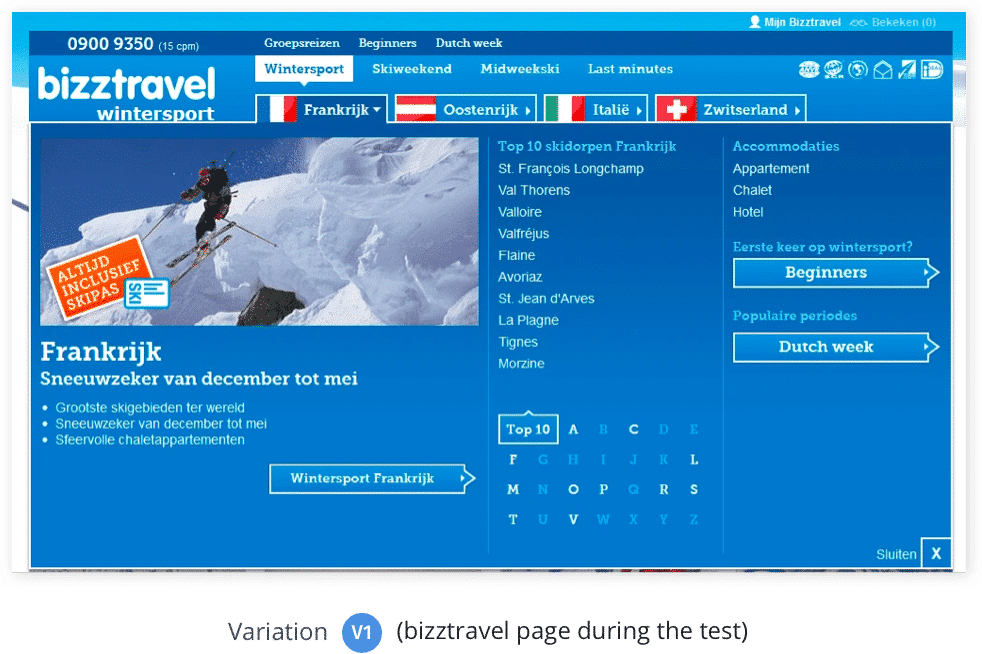
CRO for Agencies
Agencies like digital marketing, web development can greatly benefit from conversion rate optimization. By running CRO campaigns on their own sites or their clients’ sites, they can boost conversions, increase revenue, and improve ROI. This also helps retain clients and enhance business experiences.
Digital marketing agencies can pitch CRO as a service to help clients get more from existing traffic. This not only adds value to their offerings but also improves overall business impact.
Case Study: Traffic4u helped its client Djoser increase their travel bookings by 33.1%!
Djoser is a top Dutch travel agency. After analyzing its site data, Traffic4u found that adding flexibility in booking would encourage more bookings. They created a variation with a “Take an option” link, letting users reserve seats or cancel within 72 hours. The variation led to a 33.1% increase in bookings.
Read the full success story of Djoser.
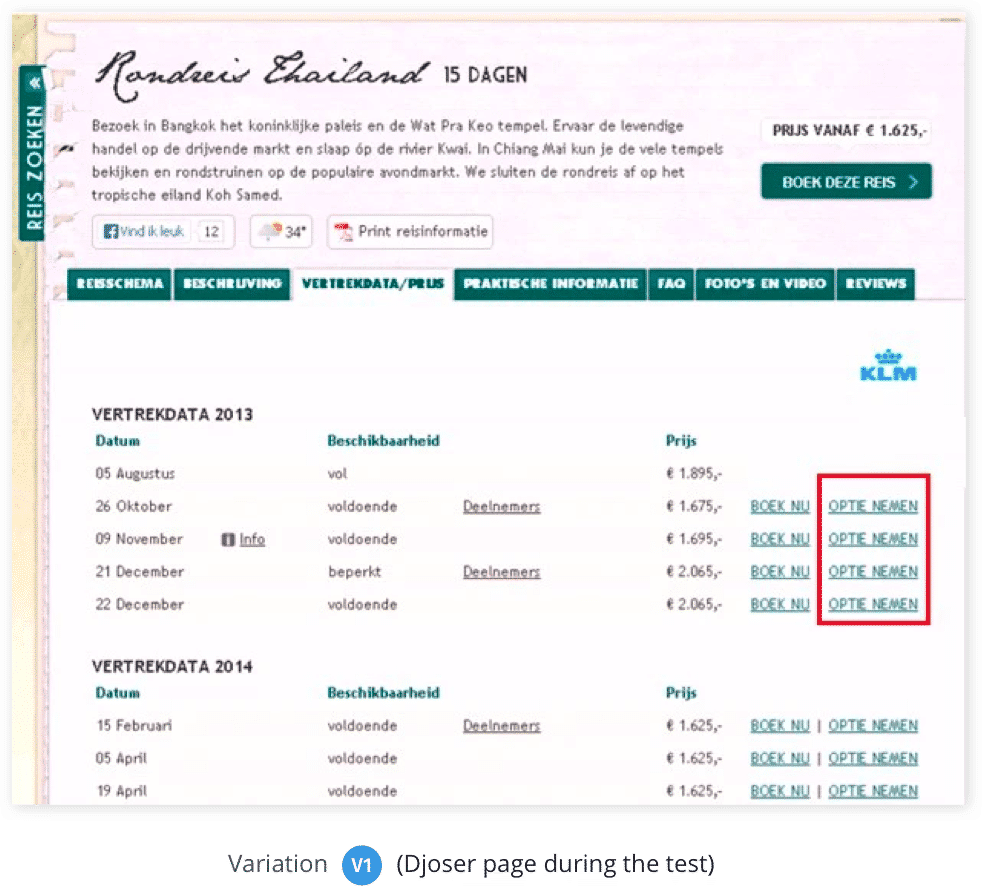
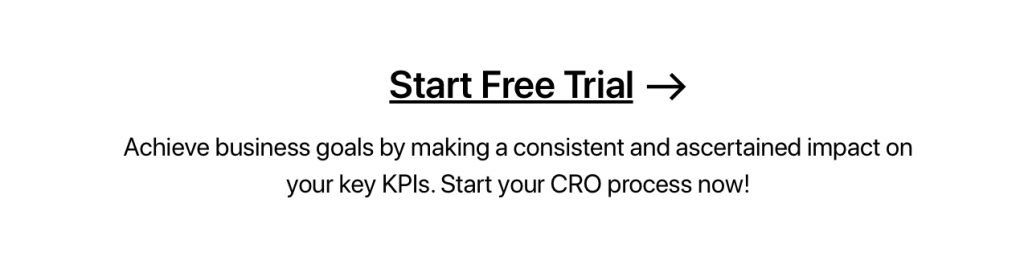
“CRO is not just a process, it’s a mindset that should be applied at every stage of your business growth.” – Lucas Kenji, Experimentation & CRO Manager at Trafilea
Source: VWO Webinar
Conversion rate optimization of mobile apps
Today, people are using numerous apps, spanning social media, music, gaming, grocery, and more. The opportunity is huge, but the competition is cutthroat. Regardless of your industry, launching an app for your business presents the challenge of making it stand out. Without fail, you must focus on app conversion rate optimization to continuously enhance user experiences and generate revenue from your app.
a. Dive into behavior of users
By leveraging behavioral analytics, such as heatmaps and session recordings, you delve into user interactions. Heatmaps reveal hotspots and areas of low engagement, uncovering user preferences and potential bottlenecks. Session recordings let you watch individual user journeys, pinpointing areas of confusion or abandonment.
b. Swift onboarding
Lengthy onboarding procedures can test users’ patience. Try simplifying the process by requesting only necessary information. This user-centric approach ensures a smooth start, increasing users’ inclination to explore the app’s offerings and subsequently boosting conversion rates.
c. Prominent CTA buttons
Making CTA buttons prominent is essential on smaller screens, where space is limited. Users may become frustrated if CTAs are not readily visible. Clear labeling, proper visual hierarchy, and strategic placement ensure that users can quickly identify and interact with CTAs.
d. Display trust elements
Demonstrating trust and security in mobile apps is crucial, given the prevalence of mobile transactions these days. Displaying trust badges, security certifications, and customer reviews reassures users about app reliability, instilling confidence in app usage. Incorporating security elements like SSL certificates and two-factor authentication further enhances user trust, ultimately encouraging app engagement.
e. Show gamification features
Integrating gamification elements into mobile apps improves user engagement and conversions. By adding rewards, challenges, leaderboards, and shared achievements, apps create a dynamic and immersive user experience, fostering a sense of achievement and community among users.
Interested in discovering more tips for app optimization, along with key metrics you must track? Explore our blog for insights on increasing app conversion rates.
9 Common conversion rate optimization mistakes to avoid
Beginners often waste time and resources on avoidable mistakes in CRO. Here are some common errors to avoid. For more details, read further on mistakes to avoid in CRO here.
a. Making changes based on opinion, not data: Don’t assume a cleaner design or another site’s successful test will work for you; always base decisions on data.
b. Writing copy that doesn’t align with business goals: Avoid well-written but irrelevant content; it must be simple, clear, and aligned with your brand’s goals.
c. Going for small tests before the big ones: Small tests won’t have a big impact; focus on larger tests with multiple optimisations to drive significant results.
d. Running too many tests or pop-ups at once: Overloading visitors with tests or pop-ups will confuse them and skew your results; limit them to keep the experience smooth.
e. Neglecting basic design elements: Avoid distractions like automatic image sliders, cheesy stock photos, and neglecting the power of product videos with CTAs.
f. Undermining CTA buttons: Don’t use weak colours, vague wording, or poor placement; your CTA buttons should be bold, clear, and easy to find.
g. Not creating a sense of urgency: Failing to show urgency, like using countdowns or limited-stock messages, can cause visitors to delay action and leave.
h. Not building trust: If you don’t display security badges, testimonials, or privacy policies, visitors may not trust you with their personal information.
i. Complicating the conversion funnel: A complicated funnel with too many steps or unclear costs will turn visitors away; simplify and personalise the process for a smoother experience.
“Maintain momentum in your CRO program. Consistency and regularity are key to ensuring the program’s success and delivering a positive return on investment.” – Chris Marsh, eCommerce CRO consultant at Dash of CX
Source: VWO Webinar
Search engine optimization conversion optimization
SEO and CRO may be different, but they complement each other. SEO drives traffic to your site, while CRO ensures visitors take the desired action. Together, they boost your website’s performance.
Benefits of SEO conversion optimization are as follows,
a. Test and identify the best versions: Failing to test SEO changes before going live can hurt rankings; continuously test key elements to boost conversions.
b. Optimize landing pages: Unoptimized landing pages hurt SEO and CRO; match keywords and improve the funnel for better action.
c. Align keywords with user intent: Don’t just target keywords; align content with user intent to increase conversions.
d. Boost website experience: Ignoring visitor insights limits optimised experiences, hurting conversions, revenue, and SEO ROI.
“When you create content, you need to serve humans. SEO only helps to optimize this content for search engines.” – Anatolii Ulitovskyi, Founder at UNmiss
Source: VWO Webinar
Conversion rate optimization for local search
Local search starts with casual queries like “near me” or “best XYZ store in the city.” Users explore online directories, check reviews, and shortlist businesses based on feedback. They may then visit the website or get directions before taking action. CRO for local search focuses on analyzing each step, identifying conversion leaks, and implementing targeted improvements.
a. Run review acquisition campaigns: Encourage customers to leave reviews using incentives or contests to boost visibility and improve your online reputation.
b. Tailor your landing page for local search: Localise content with regional keywords, testimonials, and local statistics to build trust and credibility.
c. Monitor and test website experiences: Use behaviour analytics tools to identify pain points and optimise key elements for better engagement, driving long-term success.
“When optimizing for a specific country, it’s important to consider its cultural preferences. Collaborating with someone from that country for feedback can be invaluable. There’s no need to translate or program everything from scratch—just hire a freelancer to assist with cultural guidelines.” – Vlad Bisceanu, Marketing Director at UltiMaker
Source: VWO Webinar
B2B conversion rate optimization
B2B CRO focuses on improving digital touchpoints using behavioral and analytical data to boost ROI and lead generation. With longer purchase cycles in B2B, CRO is key to creating a consistent digital experience. Even small changes in conversion rates can have big financial impacts, making optimization essential. B2B CRO efforts include content optimization, A/B testing, personalization, server-side experimentation, and website enhancements.
Here are three effective B2B CRO strategies,
a. Use a customer data platform (CDP): A CDP unifies data, uncovering insights to guide strategies that improve engagement and conversions.
b. Boost product credibility with persuasive copy: Highlight your product’s credibility with compelling copy, showcasing client stats or improvements to build trust with potential clients.
c. Implement multi-step lead generation forms: Break long forms into sections to reduce overwhelm and increase user satisfaction, leading to higher conversion rates.
“CRO is the keystone of your e-commerce or SaaS business, and personalization is the keystone of boosting a CRO performance.” – Miles Hoogwerf, Manager, Conversion Rate Optimisation at Youwe.
Source: VWO Webinar
5 Conversion rate optimization tools
Choosing the right CRO tool depends on your budget, goals, and the features you need. Here are some top conversion rate optimization tools, each with its unique features and capabilities.
1. VWO
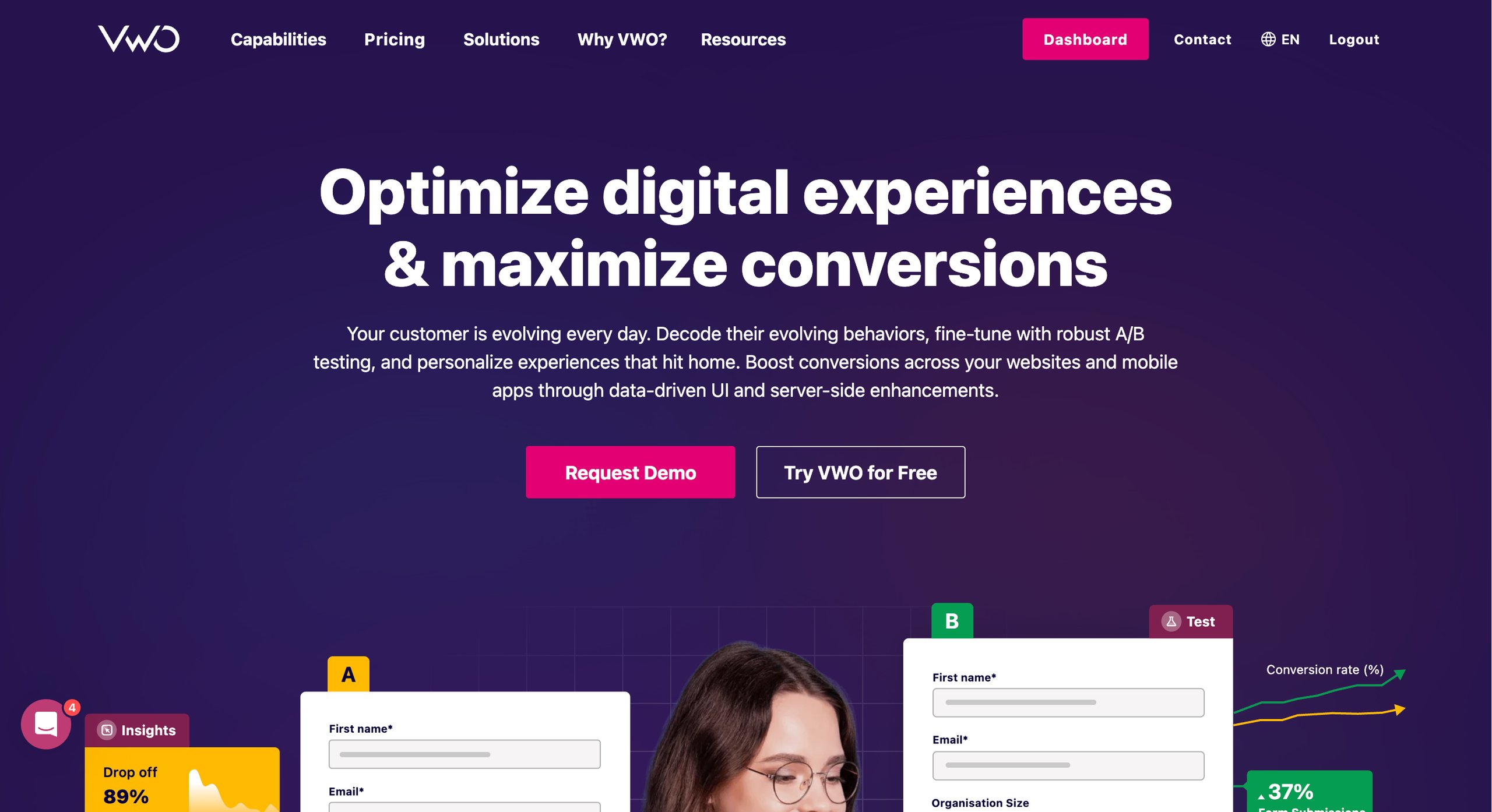
VWO optimizes every stage of the conversion process. It offers:
- VWO Insights: Provides user behavior data through heatmaps, session recordings, surveys, and funnel analytics.
- VWO Plan: Centralizes management of experimentation programs.
- VWO Testing: Supports A/B, split, and multivariate testing.
- VWO Personalize: Creates personalized user experiences. VWO covers everything from user research to personalized experiences across web and mobile.
2. Google Analytics
Google Analytics is great for tracking visitor behavior and conversions. Features like goal tracking, funnel visualization, and segmentation help identify areas for improvement and monitor CRO progress.
3. Heap Analytics
Heap offers a comprehensive view of user behavior on websites and apps. Key features include session replays, heatmaps, user journeys, funnels, and user segmentation. Heap enables data-driven decisions for better CRO outcomes.
4. TestRail
TestRail streamlines test case management and tracking. It helps organize test suites, plan test runs, and ensure software quality. It’s ideal for hypothesis prioritization and testing stages.
5. Five Seconds Test
The Five-Second Test evaluates first impressions by testing how users perceive your site in five seconds. It helps simplify design, optimize first impressions, and measure design change impact.
Choosing the right tool can significantly boost conversion rates and improve user experience. Be sure to check if a full-featured free trial is available to test the tool before committing. VWO, for example, offers a 30-day trial to explore its features.
“Integrating GA4 with A/B testing tools enhances experimentation strategies. By leveraging GA4’s audience segmentation and event tracking capabilities, businesses can refine test strategies and measure outcomes more effectively.” – Jihee Yoo, Senior Analytics Manager at Jellyfish
Source: Jihee Yoo interview
Conversion rate optimization budget and buy-in
Before you kickstart your CRO program, you must have complete clarity about the overall cost and investment required for it. Let’s take a look at some of the most crucial factors that you must consider while preparing a CRO budget.
a. Human capital cost
To achieve your CRO goals, you need to invest in human capital. This includes the time and effort of your current staff, upskilling them with CRO courses and certifications, and hiring new team members. However, creating an in-house team for CRO is usually more expensive than outsourcing the program to an agency.
b. Tool cost
Factor in the expenses for the tools or equipment required to run your CRO program. Depending on your level of CRO expertise, you can use a single, integrated tool like VWO that caters to all your optimization needs.
c. Opportunity cost
Sometimes, you lose revenue because of decisions that were not made. A/B testing is a crucial part of the CRO process that prevents you from making changes that don’t improve conversions or, worse, changes that negatively impact your conversions.
d. Experimentation regret
When conducting a test, one version usually outperforms the other, regardless of how big or small the difference may be. Experimentation regret occurs when the variant performs worse than the current version, leading to a decrease in the overall website performance.
“To run cost-efficient optimization programs, it’s crucial to test the right things. If you don’t fully understand why users are dropping off or bouncing, you might test what seems important, but other tests could yield a higher lift in conversions and revenue. Focus on understanding user behavior first.” – David Otero, CRO Specialist & Growth Expert at Speero
Source: VWO Webinar
Conversion rate optimization trends you need to know
Ignoring trends can hurt your business. Constant innovation and smart decision-making are key to success. As online visitor behaviour evolves, so must your CRO strategy. Stay ahead by embracing these trends for long-term growth.
a. Harness generative AI: Generative AI is revolutionizing CRO. Use it to run real-time experiments, summarise survey responses, and make data-driven recommendations for faster growth.
b. Focus on augmented & virtual realities: AR and VR offer personalized experiences that influence purchasing decisions. Integrating these can elevate the shopping experience and give you a competitive edge.
c. Leverage social proof: Customer reviews, testimonials, and user-generated content validate choices for visitors. Social proof builds trust, removes doubts, and boosts conversion rates.
d. Prioritize data quality: Regularly audit your data to remove inaccuracies. Clean, reliable data enhances CRO test accuracy and helps you make better decisions.
e. Research visitor behaviour: Understanding your visitors’ needs and pain points is essential. Use tools like heatmaps, session recordings, and surveys to identify and fix issues, improving the user journey.
f. Increase testing velocity: Speed is crucial in today’s fast-moving digital world. Test and iterate quickly to refine strategies and improve your site’s conversion rate. AI tools like VWO simplify this process, enabling continuous optimization.
To stay competitive in CRO, integrate the latest tech, focus on your customers, and proactively embrace emerging trends. Read our list of top 14 CRO trends to empower your business with the foresight needed to stay ahead in a dynamic market landscape.
Conclusion
CRO has become essential for improving website performance. It involves mapping optimization strategies, conducting A/B tests, and using results to enhance marketing.
CRO helps companies understand customer behaviour, perceptions and provides valuable data to shape future strategies. It is not just another tool to enhance your brand’s online performance, it’s the tool to make you stand out!
Frequently asked questions
Conversion rates can be optimized with various methods like experimentation, personalization, visitor feedback-based UI/UX change, etc.
Yes, it is worth doing conversion rate optimization as it helps in uplifting website ROI. Global brands like Dell reported a 300% increase in conversion rates from CRO initiatives like A/B testing.
SEO focuses on driving traffic through organic channels like search engines. CRO, on the other hand, is a broader initiative aimed at improving conversions across the entire funnel.
A CRO strategy is a plan to improve conversions at each funnel stage through different initiatives like SEO, experimentation, personalization, and UI/UX overhaul.
