The Region of Practical Equivalence (ROPE)
The Region of Practical Equivalence refers to a statistical concept that defines an area within which any observed differences are practically considered unimportant. Said another way, it’s like a buffer zone around a baseline value inside which changes are considered too small to matter in a real-world context, even when they attain statistical significance.
In the context of experimentation, ROPE helps determine when a difference between the control and the variation is so minor that it can be considered practically equivalent, even if it is statistically significant. This approach optimizes the testing process by quickly implementing effective changes and stopping variations that barely outperform the baseline.
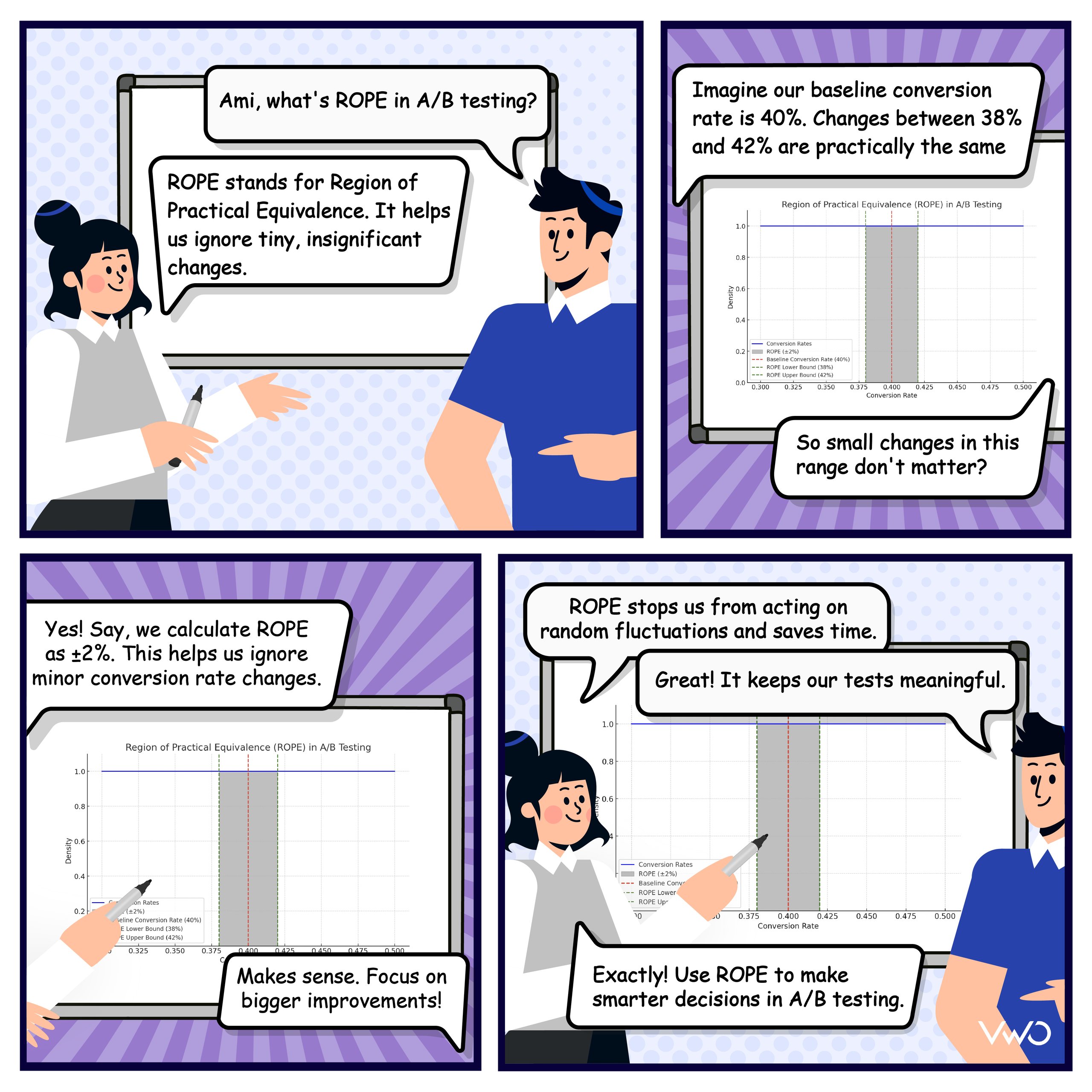
Understanding ROPE with an example
Imagine you own an eCommerce platform and your present conversion rate is 40%. You determine that for all practical purposes, any conversion rate within the range of 38% to 42% is considered equivalent to 40% for your business. (We have explained how to determine this in the following sections.)
This range—from 38% to 42%—is your ROPE.
Here’s how you do it:
Find the ends: State the lower and upper limits within which your ROPE lies. In this case, 38% and 42%.
Calculate the difference: Find the difference between these limits and the baseline. Here it is ±2%.
Normalize the Difference: Divide the difference by the baseline conversion rate (40%)
± 2 / (40%) = ± 5
So, a ±5% range around your baseline is your ROPE.
Significance of ROPE
ROPE helps save visitors from insignificant changes by closing them early. This approach helps save valuable visitors from being exposed to changes that aren’t likely to yield meaningful improvements.
As a tradeoff, you invest slightly more visitors on better variations so that you can deploy them with increased accuracy. Overall, since winning ideas are rarer and most ideas are insignificant, you save visitors significantly on average.
The wider the ROPE region, the more visitors you save. Larger ROPE means more accurate winners (in exchange for extra visitors) and early stopping of variations that do not have potential.
Minimize false positives
Random variations in your data may sometimes look like a trend of important changes. ROPE steps in to protect against these scenarios, making sure that actions will be taken only on meaningful improvements.
Suppose you’re running an ad campaign, and there is a spike in website traffic while the marketing campaign is running. If proper statistical bounds are not established, this spike may be mistaken for a successful campaign when the real reason could be other external factors such as a holiday or another viral post on social media. ROPE differentiates ‘real’ actionable improvement and random fluctuations.
Factors to consider while setting ROPE
Defaults
Reasonable default values to start with for a ROPE might be a conservative value, say, ±1%, especially if you are new to the idea. This will reduce your false positives and give you the benefits of early closing.
Later, as you start using ROPE, you can increase the ROPE value for faster closing of tests. However, doing so means you might miss out on detecting small but potentially valuable improvements within the ROPE region.
Essentially, the trade-off is between more rapid test closures and the risk of overlooking minor improvements.
Business context
Different businesses may have different thresholds for what they consider to be a meaningful change.
For example, some businesses may require very small improvements to be considered meaningful, while others may only consider larger improvements to be significant. Your understanding of what qualifies as a meaningful change in your particular context will determine the appropriate value for your ROPE.
Let’s take an example of an eCommerce retail company:
A company selling low-margin products (like groceries) might consider even a small percentage increase in conversion rate as meaningful. A 1% lift in conversion rate could translate to a significant increase in overall revenue due to high sales volume. The ROPE would be set accordingly, perhaps from -0.5% to +0.5%. Any improvement outside this range would be considered significant. In this case, small changes can be meaningful due to high transaction volume. Hence, ROPE is narrower to detect these smaller, yet significant, improvements.
But if the same store sells luxury goods, it may require a larger percentage increase in average order value to be considered meaningful. Given the higher profit margins, a smaller absolute increase in revenue might still represent a substantial improvement. A luxury goods retailer might require a 5% increase in average order value to be meaningful. Hence, ROPE would be wider, maybe from -2% to +2%, to accommodate the fact that smaller percentage changes have a lesser impact in absolute terms.
Iterative refinement
When conducting continuous testing, you may start with a much wider ROPE and then refine it based on the outcomes of the experiments. This adaptive mechanism will make your ROPE closer to the real business effect.
For example, for unoptimized and new webpages, you can aim for larger ROPE values since they should target bigger uplifts.
Optimized webpages with many visitors can benefit from small improvements as well and hence should keep a smaller ROPE.
High-traffic pages of your website should have lower ROPE values since smaller uplifts can be valuable for them. Low-traffic pages should have higher ROPE values so that early stopping can help save visitors and time.
ROPE in VWO
The good news is that ROPE has been integrated into VWO’s Statistical Engine. ROPE enables quicker decision-making since the stats engine can now recommend disabling a variation when it is unlikely to outperform the baseline. This means you will enjoy all the benefits discussed in this article and can rely on smarter and more accurate results for every test you run. Take a free trial with VWO now.