Six Pack Abs Tested Product Pricing To Increase Revenue
About Six Pack Abs Exercises
This success story has some deep ramifications for anyone selling anything online. It shows how if you’re not A/B testing your prices, you could be leaving money on the table.
Six Pack Abs Exercises (now Dr. Muscle) is a website run by Carl Juneau which provides training videos and guides on how to have a set of “rock hard abs.”
Tests run
At the time of the test, the page selling the abs workout looked like this for both Control and Variation:
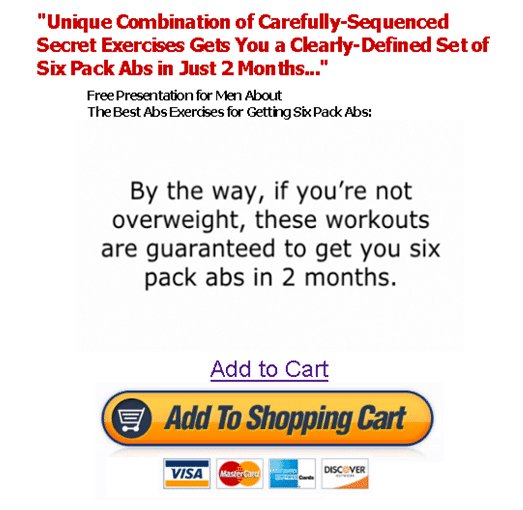
Control: On clicking Add To Shopping Cart, visitors were taken to the checkout page where the price was $19.95.
Variation: Same checkout page; the only change was that now the price was $29.95.
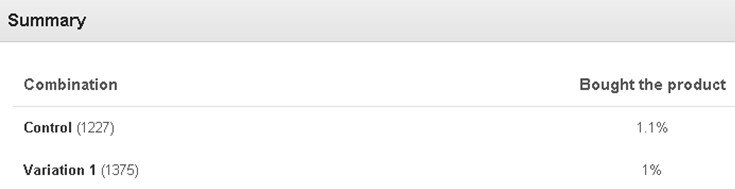
The above image shows that among 1,227 visitors who saw the original pricing ($19.95), 1.1% ended up buying.
Among the 1,375 visitors who saw the $29.95 price, 1% ended up buying.

Split testing the prices by using VWO, Carl found out that both conversion rates were statistically the same, which means customers did not differentiate between the $19.95 and $29.95 price values.
Conclusion
By A/B testing these price values, he made an extra 61.67% revenue from the Variation compared to the Control. If he were to compare them by looking at the conversion rates from 1,000 visitors each, the $29.95 price would still make him an extra 36.48% in revenue.
While it’s obvious that you should A/B test your prices to make the most revenue, what’s also important is that you’re learning the difference in how you and your customers value your offering.
To explain, I’ve used a bit of Economics.
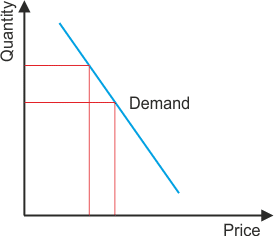
This is the traditional price elasticity curve. As the seller’s price increases, the demand for a product decreases.
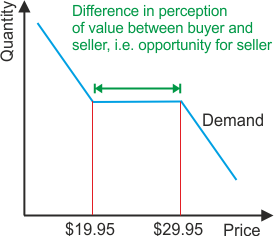
This is the price elasticity curve for this particular case. Notice the green line. There can be 2 reasons for that:
- The buyers value the Abs Training products higher than was anticipated by Carl which is why increasing the price did not decrease conversion rate.
- The buyers were indifferent to both prices and would have bought either ways.
As you’ll realize, both these situations were agreeable for SixPackAbsExercises.com and Carl. Now, all he has to do is A/B test his prices further to see if he’s still leaving any money on the table.
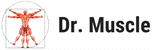
Location
Canada
Industry
Health
Impact
61.67% increase in Revenue