Multi-Armed Bandit (MAB) – A/B Testing Sans Regret
Most readers of this blog would be familiar with A/B Testing. Just as a quick reminder, A/B Testing is an experiment where a random visitor to your digital property is shown a different version than the original (also called ‘control’) in an optimizer’s quest to discover an optimal version that maximizes conversions.
For example, maybe it’s the red button that maximized clicks or maybe it’s the blue button. Who knows? Well, your A/B test would know. Systematically done A/B tests, with effective a/b testing tools, can lead to a big improvement in conversion as Moscow-based Tinkoff Bank discovered a 36% uplift.
However, in this quest to maximize conversions, there is a cost that is inevitable – while the A/B tests are in progress, a sizable portion of the traffic is routed to a losing variant directly reducing business metrics (like sales or conversions).
It is said that in an A/B test, the cost of increasing conversions is the conversions, per se. We say, ‘touche’.
Let us take the example of Jim, a UX Analyst, who works with a mobile brand that is launching its latest and greatest handset next week. To hype the demand and trigger wildfire sales, Jim decides to run flash sales on the company’s mobile app for 3 days.
Here is a rider, though – Jim is aware that the brand’s in-app navigation is poor (he ran a survey with active users to arrive at the conclusion) and visitors find it difficult to locate the product.
To improve navigation, he decides to do an experiment where he creates a variation with more intuitive navigation leading users directly into the flash sales funnel to test if this version would solve discovery issues for the new handset. In short, Jim is trying to improve a crucial KPI – percentage of sessions in which users were able to discover the new handset.
He looks at incoming data from the experiment and observes that the tweaks to in-app navigation are demonstrating strong uplift. Jim is trigger happy though – he wants to share early results with the senior leadership and get them equally excited. Just as he is about to barge into the CMO’s cabin with a copy of early trends to convince her to direct more traffic towards the new navigation, he is stopped in his tracks by a quip from a Data Scientist.
“Jim, these trends are great but are they statistically robust? Where is the significance?”
“But we don’t have time to wait for it! The sale ends in 3 days!” Jim grunts.
Who is right? Jim, who is tasked with doing the best possible within 3 days, or the data scientist who is questioning the statistical significance? Well, both are, and here’s why.
Remember the earlier quip about the cost of increasing conversions, being the conversions? Jim’s situation warrants an approach that minimizes the cost of running an A/B test. The loss of conversions due to the low-performing variation is called Bayesian regret.
Minimizing the regret is especially important in time-sensitive situations, or in cases where the cost of poor variations is so high that businesses hesitate to run A/B tests.
Since Jim is relying on a three-day window to maximize sales, he can’t wait for statistical significance and lose out on conversions, which sometimes takes weeks (or months, for low-traffic websites). If he waits for statistical significance, he won’t be able to use the results as the 3-day window will be over.
If only Jim had Multi-Armed Bandit algorithms to use, this issue wouldn’t have happened. Here’s why.
What is multi-armed bandit testing?
MAB is a type of A/B testing that uses machine learning to learn from data gathered during the test to dynamically increase the visitor allocation in favor of better-performing variations. What this means is that variations that aren’t good get less and less traffic allocation over time.
The core concept of MAB is ‘dynamic traffic allocation’ – it’s a statistically robust method to continuously identify the degree to which a version is outperforming others and to route the majority of the traffic dynamically and in real-time to the winning variant.
Unlike A/B tests, MAB maximizes the total number of conversions during the course of the test. The trade-off is that statistical certainty takes a backseat because the focus is on conversions and finding out the exact conversion rates (of all variations, including the worst performing ones).
What is the multi-armed bandit problem?
MAB is named after a thought experiment where a gambler has to choose among multiple slot machines with different payouts, and a gambler’s task is to maximize the amount of money he takes back home. Imagine for a moment that you’re the gambler. How would you maximize your winnings?
As you have multiple slot machines to choose from, you can either determine payout possibilities by taking a chance with all the machines, collecting enough data until you know for sure which machine is the best.
Doing this will reveal to you the exact payoff ratio of all the slot machines, but in the process, you would have wasted a lot of money on low payoff machines. This is what can happen in an A/B test. The alternative is to focus on a few slots faster, continuously evaluate winnings and maximize your investments over these slots for higher returns. This is what happens in the multi armed bandit approach.
Multi-Armed bandit at work
Image Source: towards data science
Exploration and exploitation
To understand MAB better, there are two pillars that power this algorithm – ‘exploration’ and ‘exploitation’. Most classic A/B tests are, by design, forever in ‘exploration’ mode – after all, determining statistically significant results is their reason for existence, hence the perpetual exploration.
In an A/B test, the focus is on discovering the exact conversion rate of variations. MAB adds a twist to A/B Testing – exploitation. Owing to the ‘maximize conversions and profit’ intent of MAB, exploitation, and exploration, run in parallel, akin to a train track – think of the algorithm exploring at a rate of many visitors per second, arriving at constantly shifting winning baselines and continuously allocating the majority of your traffic dynamically to the variant that has a higher chance of winning in that instant (exploitation).
It may sound like MAB uses heuristics to allocate more traffic to better performing variation. However, under the hood, VWO’s implementation of MAB is statistically robust. VWO uses a mathematical model to continuously update the estimated conversion rates of variations and allocates traffic in direct proportion to those estimates.
As the estimate of best-performing variation gets better, that variation gets a higher percentage of traffic. In case you’re interested in learning the mathematics of VWO’s MAB algorithm, you may want to read more about a concept called Thompson Sampling or you can request a demo from our optimization specialists to get to know more about how it works.
Over the test cycle, the algorithm balances between exploration and exploitation phases. As high performers garner more conversions, the traffic split continues to widen and reaches a point where a vast majority of users are getting served the better performing variation. MAB thus allows Jim from our example above, to progressively roll out the best version of his mobile app, without having to wait for his tests to reach statistical significance.
Exploration v/s Exploitation in A/B Testing and Bandit Selection
Why A/B Testing is better than MAB
When weighing the pros and cons of multi-armed bandit vs A/B testing, understand that they both solve different use cases due to their distinct focuses. An A/B test is done to collect data with its associated statistical confidence. A business then uses the collected data, interprets it in a larger context and then makes a decision.
In contrast, multi armed bandit algorithms maximize a given metric (which is conversions of a particular type in VWO’s context). There’s no intermediate stage of interpretation and analysis as the MAB algorithm is adjusting traffic automatically. What this means is that A/B testing is perfect for cases where:
- The objective is to collect data in order to make a critical business decision. For example: if you’re deciding the positioning of a product, engagement data on different positioning in an A/B test is an important data point (but not the only one).
- The objective is to learn the impact of all variations with statistical confidence. For example: if you’ve put the effort into developing a new product, you don’t just want to optimize for sales but also gather information on its performance so that the next time you can incorporate those learnings into developing a better product.
CityCliq saw a 90% increase in CTR after running A/B tests for product positioning and the data from this set of tests has set them up well for future tests.
Benefits of multi-armed bandit testing
In contrast, MAB is perfect for cases where:
- Efficient allocation of resources to the most promising variations is crucial, particularly when there is a concern about resource constraints. This helps reduce the opportunity cost linked to directing traffic to a suboptimal variation.
- There is no need for interpretation of results/performance of variations, and all you care about is maximizing conversion. For example: if you’re testing a color scheme, you just want to serve the one that maximizes conversions.
- The window of opportunity for optimization is short-lived and there’s not enough time for gathering statistically significant results. For example: optimizing pricing for a limited period offer.
In conclusion, it is fair to state that both A/B and MAB have their strengths and shortcomings- the dynamic between the two is complementary and not competitive.
Use cases for multi-armed bandit testing
Here are a few common real-world scenarios, where MAB has shown that it’s clearly superior to A/B Testing:
1. Opportunity cost of lost conversions is too high
Imagine you’re selling diamonds (or a car) online. Each lost conversion is probably worth thousands of dollars in lost opportunity for you. In that case, MAB’s focus on maximizing conversions is a perfect fit for your website optimization needs.
2. Optimizing click-through rates for news outlets that cover time-sensitive events
Conjuring catchy headlines was initially an editor’s job, but that is clearly passe—ask our friends at The Washington Post. The short shelf life of news pieces means that quick optimization is essential. They optimize and test headlines, photo thumbnails, video thumbnails, recommended news articles, and popular articles to drive maximum clicks inside a short window.
3. Continuous optimization
Optimizers have the ability to add or subtract multiple elements from variations and test across all simultaneously. In a traditional A/B test, there is little freedom to orchestrate multiple changes once the experiment goes live because data sanctity is sacrosanct.
4. Optimizing revenue with low traffic
If there’s not enough traffic, A/B tests can take really long to produce statistical significance. In such cases, a business may find it better to run an MAB as it is able to detect the potentially best version much earlier and direct an increasing amount of traffic to it.
Understanding the limitation of MAB: Where A/B Testing is clearly the better choice:
1. When you are aiming for statistical significance
For all their strengths, multi armed bandit experiments are not the best choice when you want to get a statistically robust winner. A/B tests are still the fastest way to statistical significance even though you might lose some conversions in the process.
2. Optimizing for multiple metrics
Mature experimentation teams track 4+ goals per experiment, as experiences are composite of primary and secondary goals. While MAB experiments work great when optimizing for one key metric, they don’t work well for multiple goals as they only factor in the Primary Goal while allocating incoming traffic.
3. Post experiment analysis
Most experimenters like to slice and dice the data gathered during an experiment to check how different segments reacted to modifications on their web properties. This analysis is possible in A/B Tests but might not be possible in MAB as sufficient data might not be available for underperforming variations.
4. Incorporating learnings from all variations (including the poor ones) into further business decisions
During the course of the test, MAB allocates most traffic to the best performing variation. This means that poor-performing variations do not get enough traffic to reach statistical confidence. So, while you may know with confidence the conversion rate for best performing variation, similar confidence may not be available for poor-performing ones. If getting this knowledge is important for a business decision (perhaps you want to know how bad is the losing variation as compared to the best one), an A/B test is the way to go.
How to implement multi-armed bandit testing
Implementing an MAB test is similar to implementing an A/B test, with only a few differences. Start by conducting research to identify the problem causing friction in the visitor journey. Create a hypothesis to achieve your goal, aligning with the problem at hand. For example, the goal of increasing form submission can solve the problem of low form conversions on your website.
When setting up the test, note that sample size, usually not a major concern for A/B tests, becomes crucial for MAB testing. Ideally, run MAB tests on high-traffic pages. Small traffic volumes may prolong the time to reach statistical significance because MAB dynamically allocates traffic based on variation performance through the exploration-exploitation tradeoff.
Hence, more time and data are needed for MAB to adjust effectively. On the other hand, higher traffic ensures a better split between variations, yielding more reliable test results.
Use our A/B test duration calculator to determine the required traffic and duration for your MAB test. Consider factors such as your website’s current traffic volume, the number of variations (including control), and the desired statistical significance.
Summing up
If you’re new to the world of conversion and experience optimization, and you are not running tests yet, start now. According to Bain & Co, businesses that continuously improve customer experience grow 4 to 8% faster than their competitors.
Watch this video if you’d like a deeper understanding of the difference between A/B testing and MAB.
Both A/B Testing and MAB are effective optimization methodologies—MAB is a great alternative for optimizers who are pressed for time and can partake with statistical significance in exchange for more conversions in a short window. Reach out to us at sales@vwo.com if you want a brush with MAB or request a demo from our MAB experts.
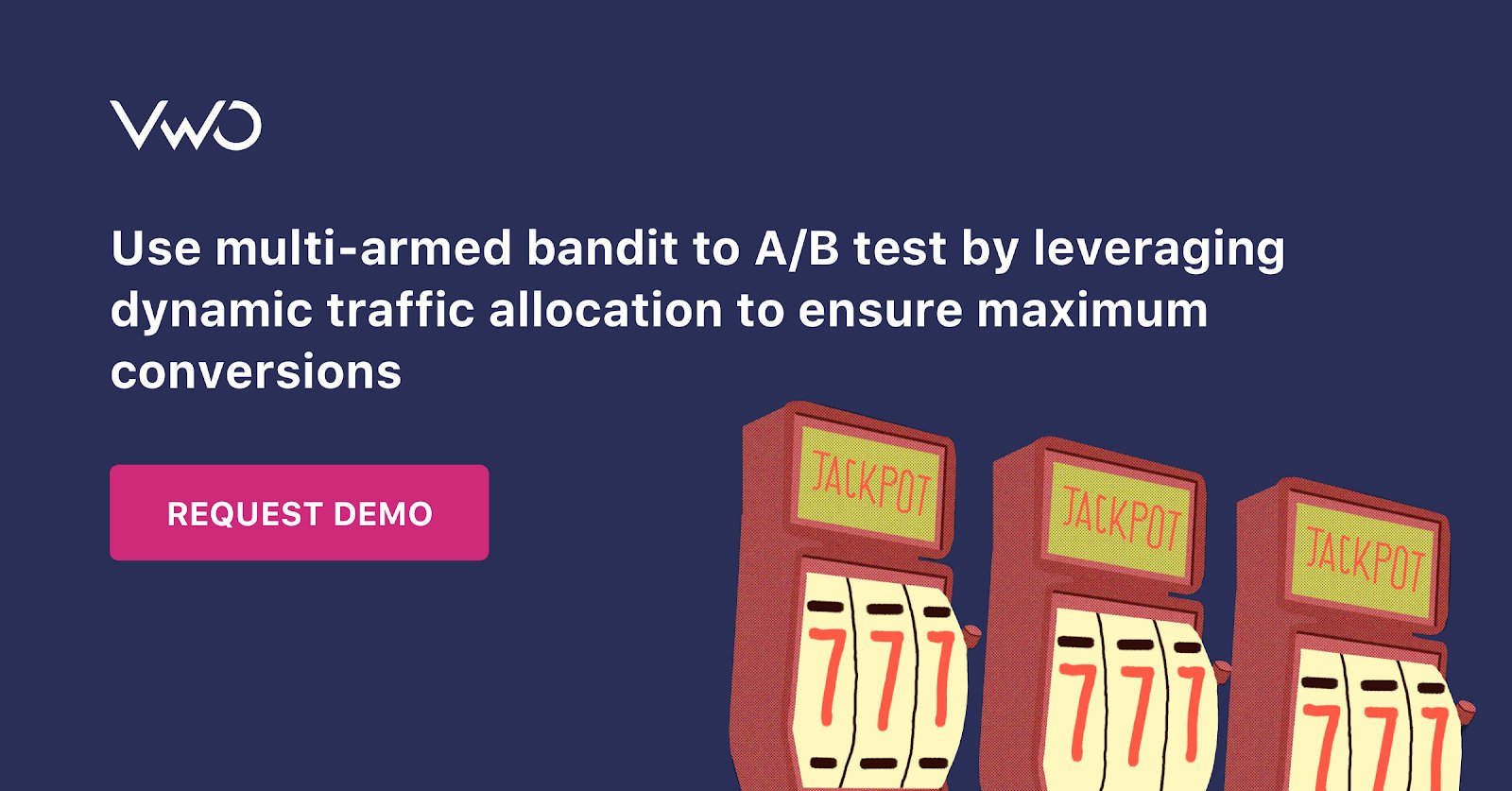
Multi-Armed Bandits (MAB) are valuable in certain optimization scenarios. In a multi-armed bandit experiment, dynamic traffic allocation to the best-performing variations ensures efficient use of resources, reducing opportunity costs by sending less and less traffic to low-performing variations. This leads to faster learning, as the algorithm adapts in real-time, maximizing conversion rates and allowing continuous optimization.
Multi-armed bandit (MAB) testing is an optimization approach where traffic is dynamically allocated to the best-performing variations to achieve a specific goal. MAB testing adjusts allocations in real time based on ongoing testing performance, making it particularly effective in high-traffic scenarios.
Before you debate multi-armed bandit vs A/B testing and decide which testing to follow, you must know the difference between them. In A/B testing, you evenly allocate traffic to variations with a fixed approach until the experiment concludes. On the other hand, a multi-armed bandit dynamically adjusts traffic to the best-performing variations in an ongoing test and allocates less and less to low-performing variations. Multi-armed bandit tests let you get to optimization quickly, while A/B testing might need longer timeframes to reach statistical significance. The choice between them depends on your experiment goals.