Thinking Like a Bayesian
Hi 👋 I am Paras Chopra, founder & chairman of VWO. Every fortnight, on this blog and on our email list, I’ll be posting a new idea or a story on experimentation and growth. Here is my 3rd letter.
I recently finished reading a book on the history of the Bayes’ theorem (appropriately called the theory that would not die) and thought you may enjoy my notes from it.
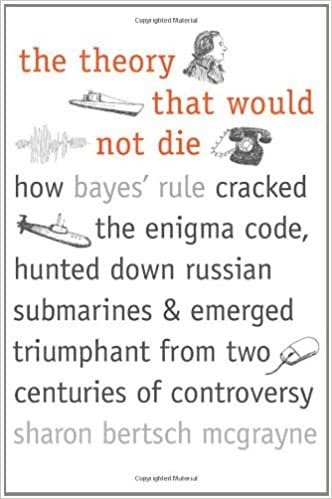
1/ Statistics is all about calculating probabilities, and there are two camps who interpret probability differently.
- Frequentists = frequency of events over multiple trials
- Bayesians = subjective belief of the outcome of events
Download Free: A/B Testing Guide
2/ This philosophical divide informs what these two camps usually bother with.
- Frequentists = probability of data, given a model (of how data could have been generated)
- Bayesians = probability of model, given the data
3/ Most often we care about the latter question and that is what the Bayesian way of thinking helps with.
For example, given that the mammography test is positive, we want to know what the probability of having breast cancer is. And given breast cancer, we usually don’t care about the probability of the test being positive.
4/ These two questions sound similar but have different answers.
For example, imagine that 80% of mammograms detect breast cancer when it’s there and ~90% come out as negative when it’s not there (which means for 10% times it comes as positive even if it’s not there).
Then if only 1% population has breast cancer, the probability of having it given a positive test is 7.4%.
5/ Read that again:
80% times the mammography test works and yet if you get a positive, your chances of having breast cancer are only 7.4%.
How is it possible?
6/ The math is simple:
- Chances that the test is positive when a patient has breast cancer = chances of detecting breast cancer when a patient has it * chances of having breast cancer in the first place = 80% * 1% = 0.8%
- Chances that test is positive when a patient does NOT have breast cancer = chances of detecting breast cancer when a patient DOESN’T have it * chances of NOT having breast cancer in the first place = 10% * 99% = 9.9%
Now, the chances of having breast cancer on a positive mammogram are simply:
% times you get a positive mammogram if you have breast cancer / % times you can get a positive mammogram.
We calculated these numbers above, so this becomes
0.8%/(0.8%+9.9%) = 7.4%.
Voila! So even if a test works 80% of the times, it may not be very useful (if population incidence rate is low, which is 1% in this case). This is why doctors recommend taking multiple tests, even after a positive detection.
7/ When you understand Bayes’ theorem, you realize that it is nothing but arithmetic.
It’s perhaps the simplest but most powerful framework I know. If you want to build a better intuition about it, I recommend reading this visual introduction to Bayes’ theorem (which also contains the breast cancer example we talked about).
Download Free: A/B Testing Guide
8/ The key idea behind being a Bayesian is that *everything* has a probability.
So instead of thinking in certainties (yes/no), you start thinking about chances and odds.
9/ Today, Bayes’ theorem powers many apps we use daily because it helps answer questions like:
- Given an e-mail, what’s the probability of it being spam?
- Given an ad, what’s the probability of it being clicked?
- Given the DNA, is the accused the culprit?
- And, of course, given the data, is variation better than the control in an A/B test? (FYI – we use Bayesian statistics in VWO)
10/ That’s it! Hope you also fall in love with the Bayesian way of looking at the world.
If you enjoyed reading my letter, do send me a note with your thoughts at paras@vwo.com. I read and reply to all emails 🙂