Building great products is about continuously testing, learning, and adapting. The most successful products evolve through a cycle of experimentation, feedback, and iteration.
In today’s fast-moving landscape, where user behaviors shift and market conditions change rapidly, experimentation is a fundamental approach to shaping products that resonate with users.
Welcome to the thirteenth post of CRO Perspectives, where we speak with experts in experimentation and optimization to stay ahead of the latest trends, uncover key learnings, and refine our strategies.
In today’s post, we speak with Dom Light, who will shed more light on experimentation in product management and its many facets, offering valuable insights into how teams can leverage testing to build better, more impactful products.
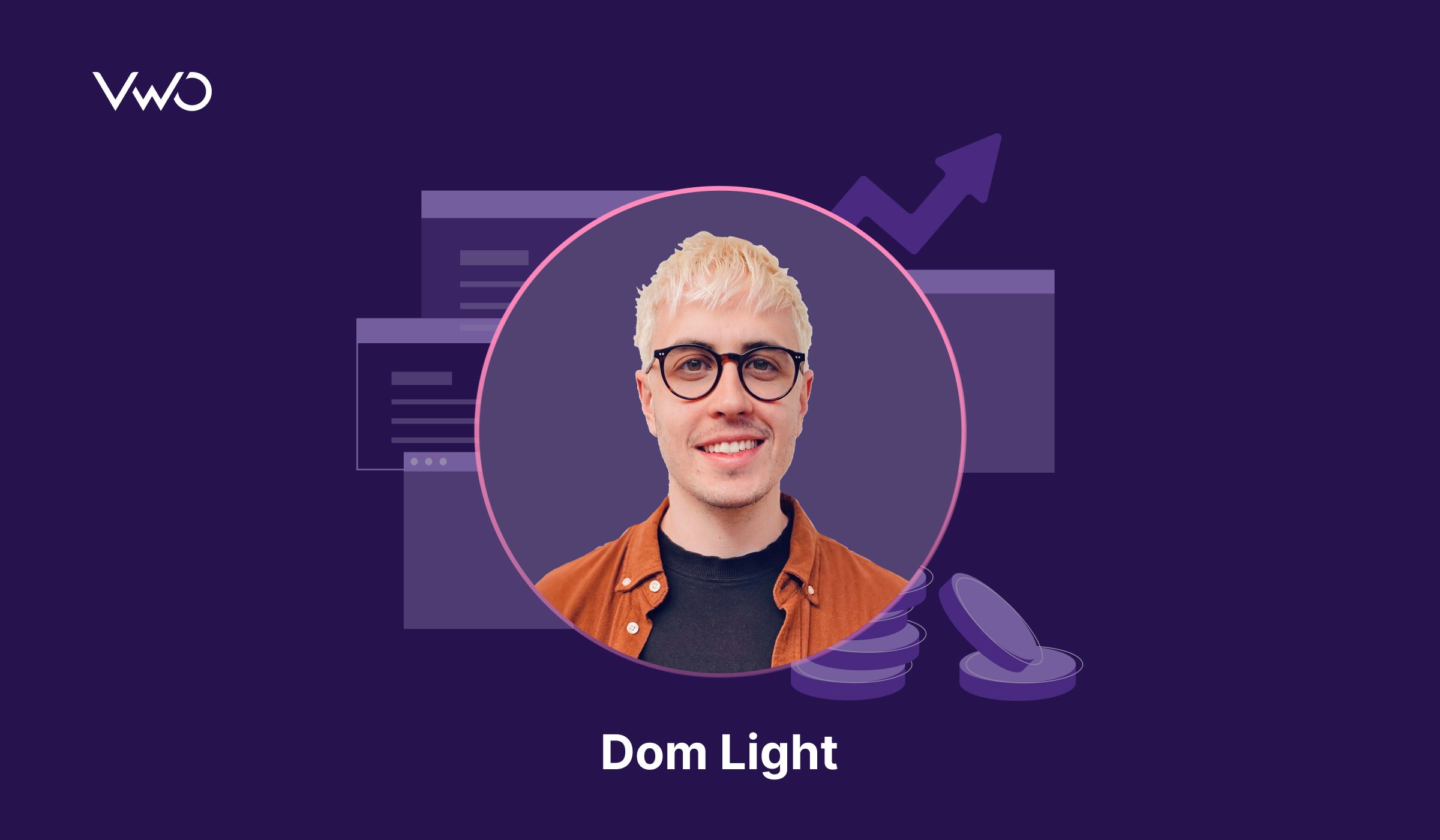
Leader: Dom Light
Role: Product Manager at Xero
Location: New Zealand
Speaks about: Product strategy • Product management • Experimentation
Why you should read this interview
Dom Light is passionate about using data and experimentation to build better products. At Xero, he focuses on optimizing the Payments experience within Xero Invoicing, ensuring small businesses can get paid faster and more efficiently.
Balancing user needs, product performance, and business goals, Dom believes experimentation is more than just testing—it’s about making informed decisions that drive real impact.
In this interview, he shares insights on how experimentation shapes product strategy, the role of AI on product management, the future of experimentation, and more.
Essential skills to excel in product management
Product management is an incredibly broad discipline, and often, it really does feel like you’re required to excel in a wide range of skills. While versatility is essential, one area I believe is becoming increasingly critical is data literacy and analysis.
This doesn’t mean you need to understand every detail of the data warehouse or backend systems behind your product analytics. However, you must have a strong grasp of where data originates across the customer lifecycle and how it connects to key moments of decision-making.
Spending time analyzing this data—segmenting it by customer properties or behavioral patterns—empowers product managers to identify friction points, generate hypotheses for experimentation, and evaluate outcomes. This iterative process helps build stronger, evidence-based decisions about product direction.
Importantly, this skill aligns with the evolving role of product management. Gone are the days when product managers could confidently launch a feature and move on without thorough validation. Today, the ability to precisely measure how changes affect key business outcomes is non-negotiable. Product managers who master data literacy to inform, iterate on, and measure impact stand out as excellent, not just good.
Having near real-time access to customer behavior and event data is crucial—not just for evaluating the success of products and experiments but also for developing a deeper, data-driven understanding of your customers, often beyond what they consciously recognize about themselves.
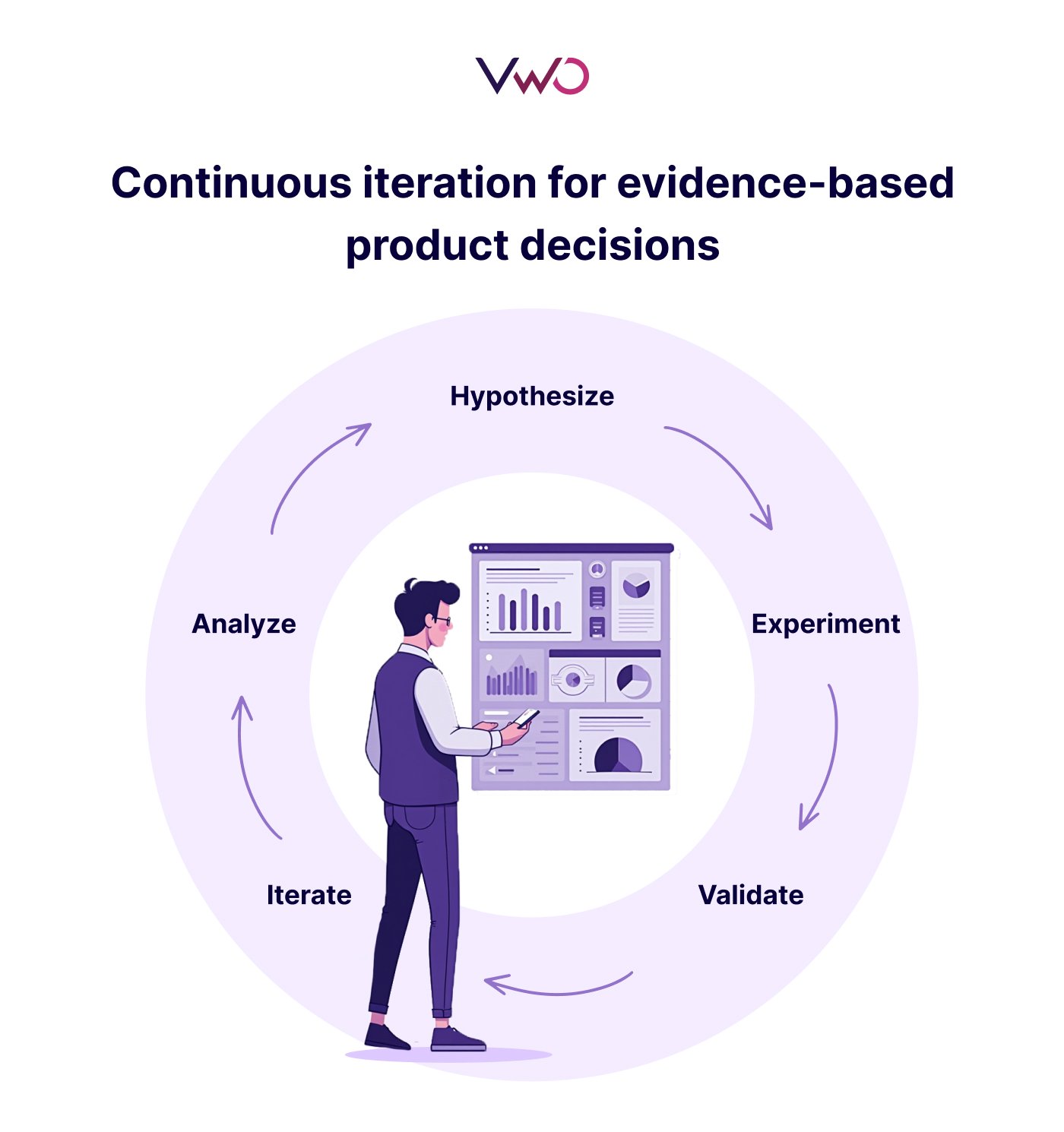
How to align experimentation with business goals
Aligning experimentation programs with overall business objectives can be a challenging task, but it’s crucial for driving meaningful impact. This alignment starts at the organizational level, with clearly defined business outcomes and accountability for the teams tasked with delivering them. While OKRs are a popular tool, they often falter when there’s a disconnect between the driver metrics a team can influence and the broader outcomes they need to achieve.
The foundation of a successful experimentation program lies in setting up the right tooling and event tracking to track these driver metrics. Teams must be able to measure each stage of the conversion funnel they’re optimizing for. Without a clear, measurable view, accurately assessing the impact of experiments is nearly impossible. To achieve this, teams should work backward from the desired business outcome, ensuring all data points and metrics tie into the ultimate goal.
At Xero, our experiments focus on improving key user behaviors that drive business outcomes. For example, in our invoicing and payments space, we track metrics like invoice open rates, online payment conversion rates, and time-to-payment.
For onboarding and adoption, we might look at activation rates – how many users complete key setup actions – and engagement metrics, such as how frequently they interact with core product features within the first few weeks.
We also incorporate qualitative insights alongside behavioral data to understand user friction points. The key is ensuring that every experiment contributes to a measurable business goal, whether that’s customer retention, revenue impact, or operational efficiency.
If the data points don’t exist, work with engineering to ensure the right level of eventing is in place—it’s well worth the investment.
This approach also helps focus efforts in the right areas. Early on, my team faced this challenge during our transition to an experimentation-driven framework. We initially targeted the top of the funnel, focusing on onboarding new organizations to use our invoicing product.
While this wasn’t a bad strategy in isolation, it led us down the path of proposing features better suited for achieving product-market fit rather than funnel optimization. Recognizing this, we shifted our attention to the bottom of the funnel, where we had greater control over outcomes. The results spoke for themselves, and it’s a strategy we’ve stuck to ever since.
By linking experiments to measurable outcomes and narrowing focus, teams can avoid the common pitfall of spreading their efforts too thin, ensuring they work on the right levers for business success.
Learnings from recent product launches
One of the most fascinating aspects of experimentation is embracing the possibility of being completely wrong—it’s part of what makes the process so rewarding. Experimentation often challenges our strongly held assumptions in unexpected ways. And it’s not always as straightforward as a “failed” experiment; sometimes, the insights are much subtler.
Recently, we ran a series of experiments on a new payer experience that we believed would perform exceptionally well for a specific B2C customer segment. In fact, our confidence was so high that some stakeholders questioned whether testing was even necessary or suggested restricting the test solely to that target group.
Still, we chose to proceed with a broader four-week experiment. While the results were undeniably positive, they revealed something surprising: the targeted B2C segment actually experienced a decline in conversion. Instead, we saw a significant uplift from a previously excluded B2B segment that we had deemed irrelevant at the outset.
This unexpected outcome boosted our overall conversion metrics and gave us a fresh perspective on an overlooked segment. The insights from this experiment not only opened up new opportunities for targeting but also reinforced the value of testing, even when the outcome seems “obvious.” It’s a great reminder that every experiment carries the potential to broaden our understanding in unexpected ways.
Making experimentation a core process
The Sonos example is an important reminder of why being customer-centric and product-led remains critical in the tech industry. They introduced a lot of friction into one of their key user channels by adding unnecessary steps, seemingly without a strong focus on quality or usability. This led to widespread frustration, and they’ve suffered with brand reputation since.
Ironically, experimentation could have helped Sonos avoid many of the issues they faced with their app relaunch. Additionally, iterative rollouts through experimentation allow teams to gain confidence in larger updates and provide better control when adjustments or rollbacks are needed.
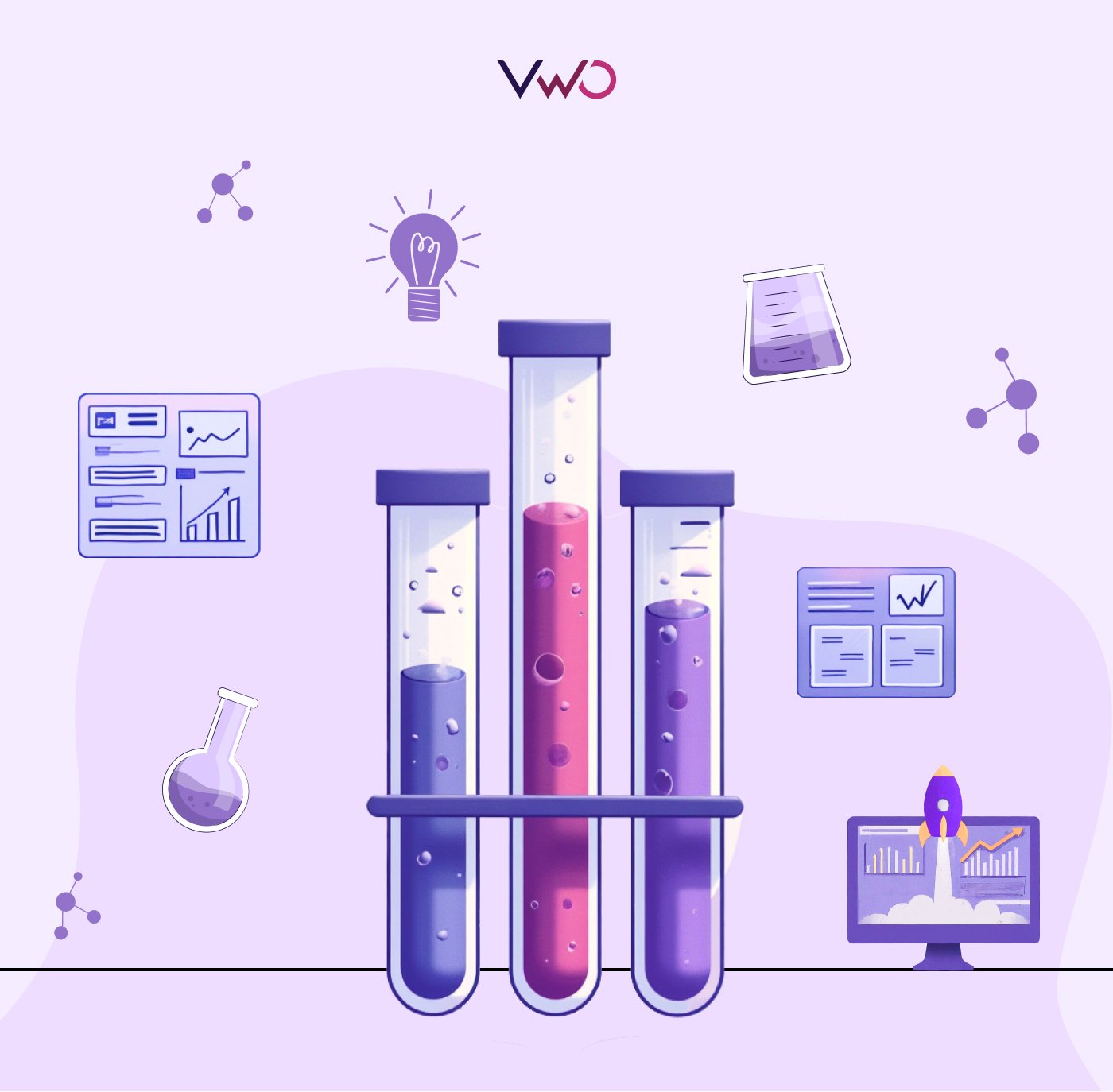
I’ve experienced firsthand how even well-tested experiments that reduce customer friction can still face backlash upon wider rollout. While it’s easy to attribute this to change aversion, it often comes down to communication. Ensuring customers understand why a change is being made and clearly articulating the benefits can help alleviate resistance and create a more positive adoption experience.
Many teams treat experimentation purely as an optimization tool, but it’s far more than that. It’s a powerful way to deeply understand user behavior in ways that traditional research methods, like user interviews, often can’t capture. It also plays a crucial role in validating key assumptions before changes are rolled out at scale.
AI’s impact on product management
AI is undoubtedly transforming product management, redefining workflows, and helping amplify both productivity and strategic thinking. For me, integrating AI tools like ChatGPT and Google Gemini into my workflow is not just about efficiency but also about improving the effectiveness of collaboration and decision-making processes.
One key way is in workshop planning and facilitation. Crafting effective workshops requires understanding team dynamics, tailoring activities to specific goals, and managing discussions to foster alignment. Here, AI can assist by generating tailored agendas, suggesting relevant frameworks based on meeting objectives, and capturing post-workshop outcomes efficiently.
AI has also been incredibly impactful in capturing and synthesizing product discussions. Tools that transcribe and summarize meetings free me from multitasking during stakeholder reviews or cross-functional syncs. This ensures I can focus on listening actively, facilitating deeper conversations, and building stronger relationships—all critical soft skills in product management.
By blending AI-powered tools with human-centric soft skills, I can drive better outcomes—whether that’s a well-run workshop that achieves buy-in or a sharper focus on the “why” behind data insights.
AI doesn’t replace the art of product management; it enhances my ability to work smarter, focus on high-value tasks, and navigate the complexities of leading cross-functional teams effectively.
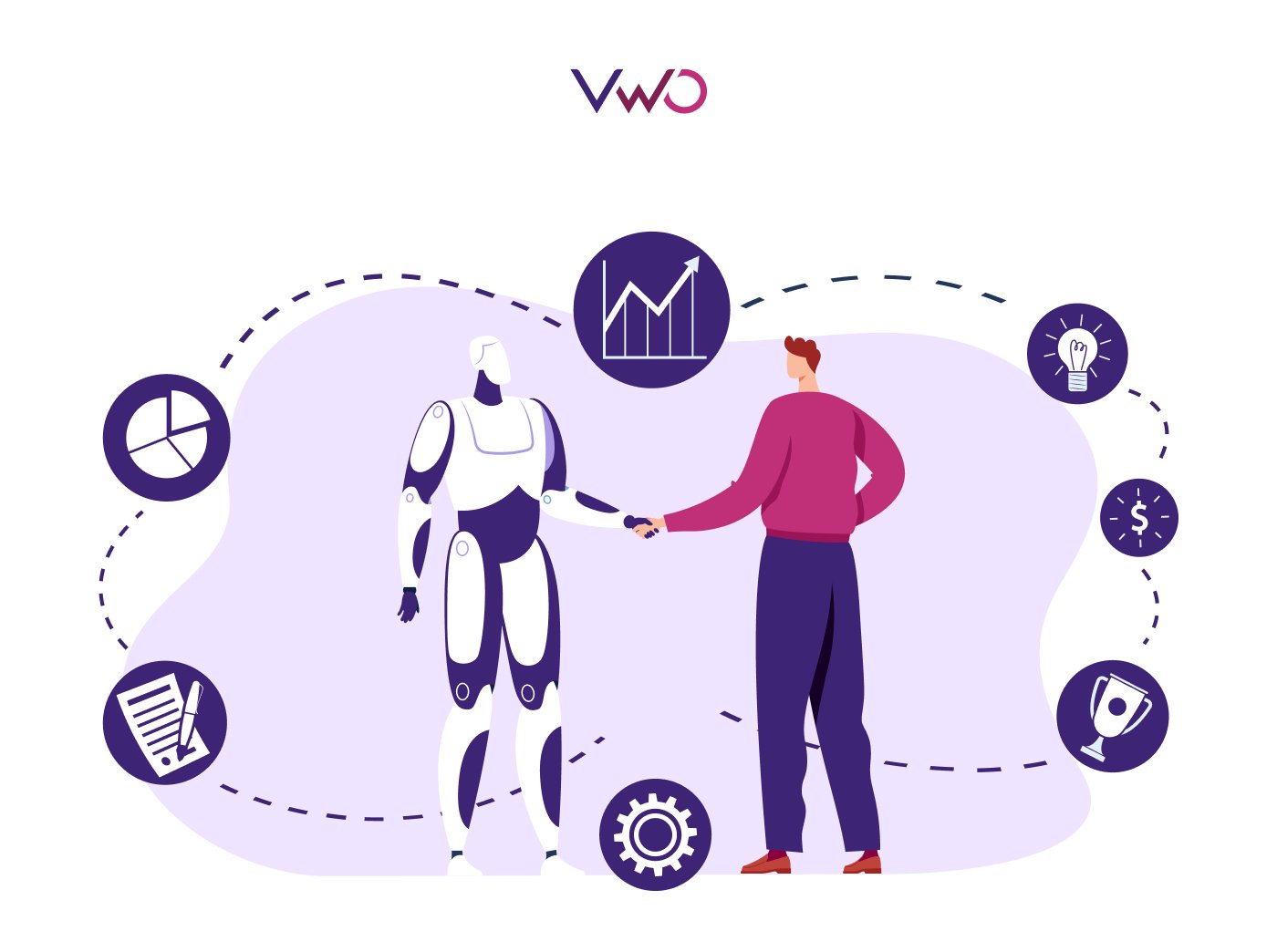
Role of product managers in ensuring data privacy
From my perspective, privacy considerations must begin at the inception of a feature or product. Building analytics and data collection capabilities should go hand in hand with asking critical questions like:
- “Do we really need this data?”
- “How would I feel if this were my data being collected, stored, or shared?”
- “Is this being stored in a fully anonymized way?”
These questions shift the focus from simply meeting technical or legal compliance to truly understanding the human impact of data collection. A privacy-first mindset ensures we build products that respect user trust rather than erode it.
In practice, this means close collaboration with legal, compliance, and security teams. At Xero, for example, our adherence to ISO data compliance standards is baked into how we work, ensuring we meet high privacy and security thresholds at every stage of the product life cycle. However, these practices often start and end with product managers. It’s our job to ensure that these considerations are fully embedded, from discovery through implementation and beyond.
The conversation becomes even more complex with the advent of large language models (LLMs) and machine learning. While organizations may have the right to collect and analyze data for product optimization, there’s a growing ethical question around using this data to train AI models. Just because we have the technical and legal ability to do something doesn’t mean we should – especially when trust is such a critical currency in industries like fintech.
Ensuring data privacy is a fundamental responsibility of product managers. While our role often focuses on collecting and analyzing data to uncover trends and opportunities, we must balance this with a deep commitment to ethical data handling and user trust.
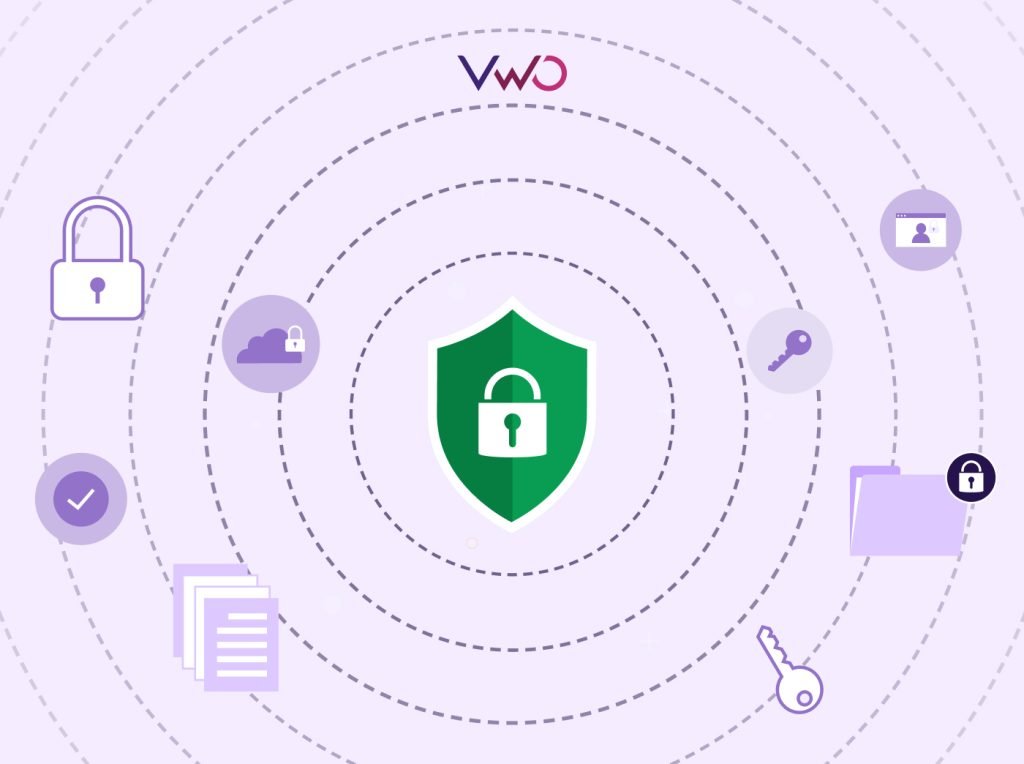
How tech vendors can support fast-growing businesses
A few months ago, I heard a great quote about experimentation and A/B testing: “It doesn’t get easier, it just gets faster.” That perfectly captures the value of tools like VWO, which help businesses accelerate testing and iteration without the need for extensive infrastructure. Increasing the speed at which product teams can deploy, analyze, and act on experiments allows companies to stay ahead of the competition by making data-driven decisions faster.
To truly support fast-growing businesses, these tools need to go beyond basic test execution and provide a comprehensive view of user behavior. Seamless integration with analytics platforms like Statsig, Amplitude, or Mixpanel ensures that experimentation isn’t just about isolated test results but contributes to a broader understanding of customer interactions across the entire funnel.
Ultimately, the more technology vendors can reduce friction in setting up, running, and analyzing experiments, the faster businesses can iterate and refine their products based on real user behavior.
Analyze large volumes of session recordings, survey responses, and color-coded heatmaps to uncover how users interact with your website. You can also automate hypothesis generation, variation creation, and test setup with VWO’s AI-powered assistant.
The future of experimentation—what’s next?
Experimentation has proved that it’s here to stay, not just as a marketing or CRO tool, but as a valuable asset to any product and engineering team in making decisions and gaining confidence in our customer segments. As technology advances, experimentation is becoming more automated, scalable, and deeply integrated into the product development process.
Looking ahead, I also see CRO evolving beyond just conversion metrics to focus on broader business outcomes like customer retention, engagement, and lifetime value. As companies mature in their experimentation culture, CRO will likely become more integrated into decision-making at all levels, moving from isolated A/B tests to a more holistic, data-driven approach to product and growth.
I see the most effective CRO function sitting within product teams rather than as a standalone department. This ensures that optimization efforts are closely tied to product-led outcomes, customer experience improvements, and long-term growth rather than short-term conversion wins.
That said, CRO can also have strong ties to marketing, particularly for top-of-funnel optimizations like acquisition and landing page performance. In some cases, a hybrid model, where CRO experts sit within the product but collaborate closely with the marketing and data team, can work well, ensuring insights are leveraged across the organization.
AI-driven experimentation is a key trend, not only streamlining A/B testing but also automating hypothesis generation, predicting test outcomes based on historical data, and refining models with each experiment run. This continuous learning approach enhances how product teams understand customer behavior, enabling faster and more effective decision-making. Tools like Statsig and VWO are already embedding AI to reduce manual effort and make experimentation more actionable, and I can’t wait to see where they go next.
Alongside AI, the shift toward server-side and full-stack experimentation is transforming how companies test and deploy changes. Moving beyond simple UI adjustments, this approach allows teams to experiment with backend performance, pricing models, and AI-driven personalization, ensuring seamless and data-driven iterations.
Uber, for example, has leveraged full-stack experimentation to optimize key operational components, such as fare calculations and driver dispatch, while maintaining a stable user experience. As businesses continue adopting feature flagging and controlled rollouts, experimentation is no longer just a phase in product development—it’s a fundamental capability that drives confident decision-making and minimizes risk.
Conclusion
We hope you gained valuable insights and answers related to product management from Dom Light.
As Dom highlighted, having a platform that goes beyond basic testing is crucial for driving business growth. With its comprehensive features, VWO stands out as the ideal choice. Whether you’re just starting with experimentation or looking to scale using advanced and secure features, VWO has you covered.
Speak to our team to explore all the features and learn how your business can benefit from our comprehensive experience optimization platform.