A/B Testing Is Like Chess
Hi 👋 I am Paras Chopra, founder & chairman of VWO. Hope you are finding my fortnightly posts outlining a new idea or a story on experimentation and growth useful. Here is the 4th letter.
The rules of chess are easy to remember: a pawn moves one step forward, the queen can go anywhere and the end goal of the game is to protect the king. Once you remember the rules, the game is easy to set up and fun to play.
But being easy in principle doesn’t mean it’s also easy in practice. Truly mastering chess can take decades of daily practice and requires memorizing thousands of nuances about opening moves, closing moves, and opponent strategies.
Download Free: A/B Testing Guide
A/B testing is very similar to chess in that sense.
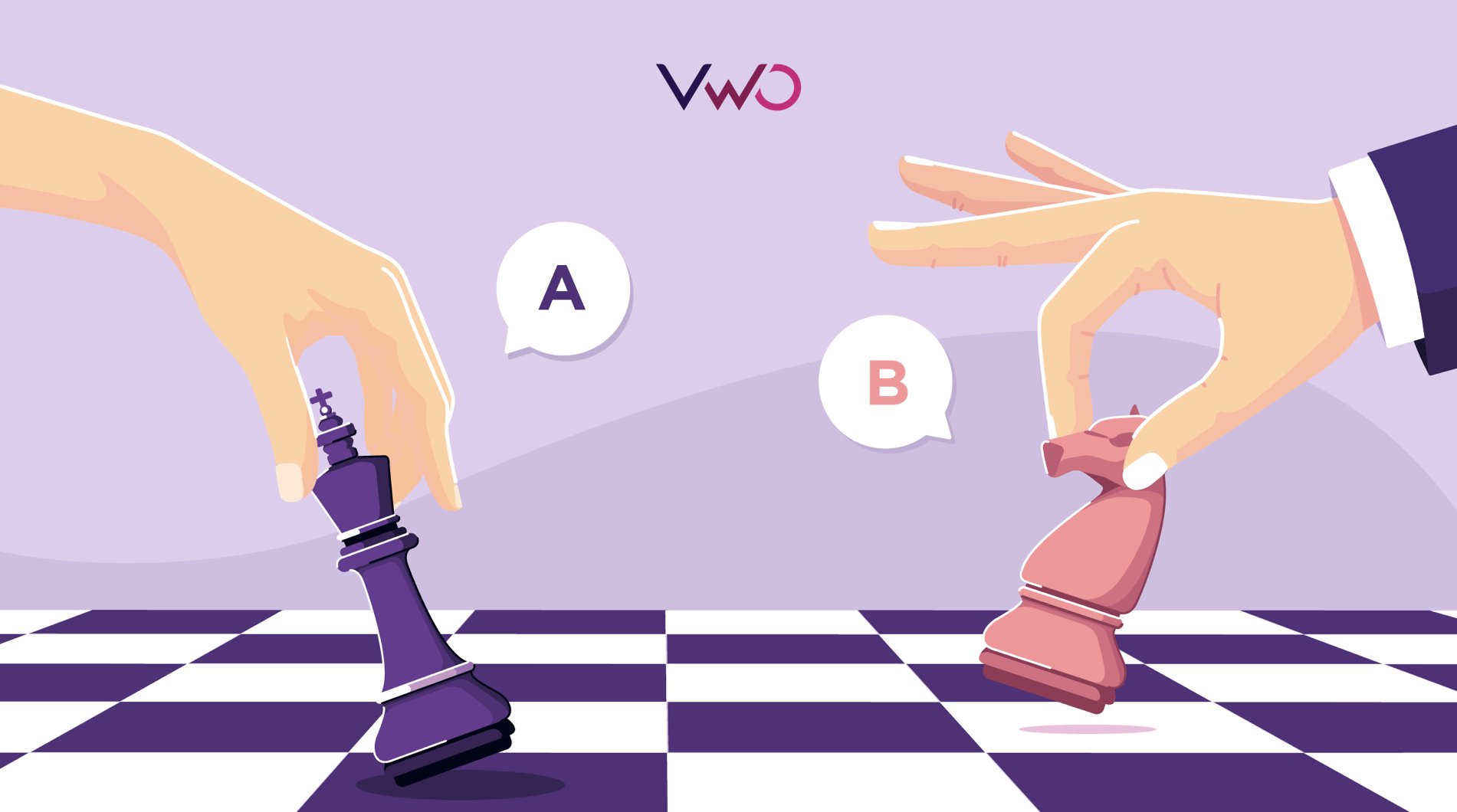
In principle, A/B testing is simple: you have two variations, each of which gets equal traffic. You measure how they perform on various metrics. The one that performs better gets adopted permanently.
In practice, however, each word in the previous paragraph deserves a book-length treatment. Consider unpacking questions like:
1/ What is “traffic” in an experiment?
Is it visitors, users, pageviews, or something else? If it is visitors, what kinds of visitors? Should you include all visitors on the page being tested, or should you only include the visitors for whom the changes being tested are most relevant?
2/ What is “measurement” in an experiment?
If a user landed on your page and did not convert, when do you mark it as non-conversion? What if the user converts after you’ve marked it as non-conversion? How do you accommodate refunds? If different user groups have markedly different conversion behavior, does it even make sense to group them during measurement? If you group them, how do you deal with Simpson’s paradox?
3/ What types of “various metrics” should you measure?
Should you have one metric to measure the performance of variations, or should you have multiple? If you measure revenue, should you measure average revenue per visitor, average revenue per conversion, 90th percentile revenue, frequency of revenue, or all of them? Should you remove outliers from your data or not?
Download Free: A/B Testing Guide
4/ What does “perform better” mean?
Is 95% statistical significance good enough? What if it is 94%? What if the new variation is not performing significantly better but feels it should? Do you take a bet on those? What if one metric improved but another that should have improved as well actually became worse? How real is Tyman’s law, which states that extreme improvements are usually due to instrumentation effort?
For the skeptic, these questions may seem like a needless pedantic exercise. But, without rigor, why bother doing A/B testing in the first place?
Nobody likes their ideas, and efforts go to waste, so we latch onto any glimmer of success we see in our A/B tests. It’s relatively easy to get successful A/B tests because it presents many avenues for misinterpretation to a motivated seeker. It’s only human to be biased.
But because of this lack of rigor in A/B testing, many organizations that get spectacular results from their A/B tests fail to see an impact on their business. Contrast this with organizations who take their experimentation seriously: Booking.com, AirBnB, Microsoft, Netflix, and many other companies with a culture of experimentation know that getting good at A/B testing takes deliberate commitment.
So, next time someone tells you that A/B testing doesn’t work, remind yourself that it’s like saying chess is a boring game just because you’re not good at it.
If you enjoyed reading my letter, do send me a note with your thoughts at paras@vwo.com. I read and reply to all emails 🙂