A/B Test Statistical Significance Calculator
Want to run an online A/B test? Calculate its significance with our Bayesian-powered calculator built for experimentation, CRO, and UX Optimization.
P-Value
0Significant?
YesDer P-Wert ist x.xx. Ihre Ergebnisse sind also statistisch signifikant!
Super, Sie verstehen, wofür der p-Wert steht! Leider sind die meisten Menschen nicht in der Lage, p-Werte richtig zu interpretieren. Aus diesem Grund haben wir VWO SmartStats entwickelt, eine Bayessche Statistik-Engine, die auf einen p-Wert vollständig verzichtet.
Leider bedeutet dies nicht der p-Wert. Keine Sorge, die meisten Leute sind nicht in der Lage, p-Werte richtig zu interpretieren. Aus diesem Grund haben wir VWO SmartStats entwickelt, eine Bayessche Statistik-Engine, die auf einen p-Wert vollständig verzichtet.
P-Value
(Bereich von 0-1)0.334
Significance
No
What is statistical significance?
Statistical significance quantifies whether a result obtained is likely due to chance or some factor of interest. You can utilize a significance calculator to confidently evaluate and interpret your results. The data-driven approach empowers you to make informed decisions for optimization and ultimately achieve your desired outcomes.
Different approaches to calculate statistical significance
The two commonly used approaches are Frequentist and Bayesian, which power different test statistics calculators. Here is a brief information on each.
Frequentist
Frequentist statistics involves examining the frequency of events. It looks at how often something happens in a given situation.
Bayesian
Bayesian statistics is all about changing your opinion. It starts with an initial belief called the prior, which comes from domain expertise. Then, you gather evidence to support or challenge that belief. After examining the evidence, your opinion should be updated based on the new information you’ve acquired.
How do we calculate statistical significance?
Here is how you can calculate statistical significance using the Frequentist and Bayesian approaches.
Frequentist approach
The Frequentist approach to statistical significance is based on the p-value. To determine the p-value using the Frequentist approach, you will need the following key pieces of information:
- The mean difference between the two hypotheses
- The standard deviation of the difference between the two hypotheses
- The sample size of each hypothesis
Once you have gathered this necessary data, you can easily compute the p-value using a significance testing tool or significance level calculator. If the p-value you get is 0.05, the probability of the variation is 5%.
Bayesian Approach
In this, you do statistical significance calculation through posterior probability. The posterior probability considers the available data and represents the probability of hypothesis A or B being true. The approach takes into account both prior beliefs and the evidence observed in the data.
To calculate the posterior probability, Bayes' theorem is employed. Bayes' theorem is a mathematical formula that combines the prior probability of a hypothesis with the likelihood of the data to derive the updated or posterior probability.
A posterior probability of greater than 95% is considered to be strong evidence in favor of the hypothesis.
Know more about the mathematical formula for Frequentist and Bayesian approach in a whitepaper that we wrote on Bayesian A/B testing at VWO.
Darum verwenden wir Bayes'sche Statistik
Intuitive Testberichte
At VWO, we recognize that non-statistical users often misinterpret the frequentist p-value as a Bayesian posterior probability, which leads to incorrect conclusions about the superiority of one variation over another. To address this issue, we developed the industry's first Bayesian statistical engine.
Our Bayesian statistical engine provides users with easily understandable results, eliminating the risk of making mistakes while conducting A/B tests on revenue or other crucial key performance indicators (KPIs). By adopting a more intuitive approach, we ensure that our users obtain accurate insights from our A/B test statistical significance calculator.
With our solution, you can have confidence in the statistical significance of your results, making informed decisions to optimize your testing, revenue generation, and overall user experience. Say goodbye to misinterpretations and embrace the power of the Bayesian A/B test calculator for reliable and actionable A/B testing outcomes.
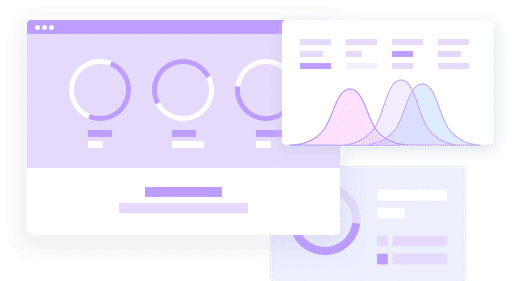
Keine Mindestgröße bei Stichproben erforderlich
VWO SmartStats basiert auf Bayes'scher Inferenz, die im Gegensatz zu einem frequentistischen Ansatz keine Mindeststichprobengröße benötigt. So können Sie A/B-Tests auch auf Teilen Ihrer Website oder Apps durchführen, die nur wenig Traffic erhalten. Wenn Sie jedoch mehr Traffic auf Ihre Tests bekommen, kann VWO Ihre Conversion Rates mit größerer Sicherheit bestimmen, sodass Ihre Testergebnisse genauer werden.
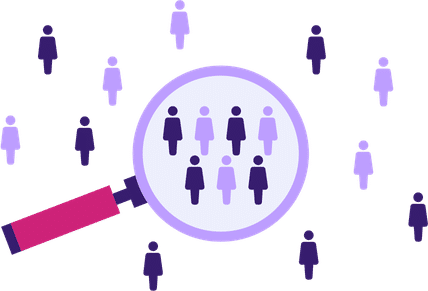
Kommen Sie schneller an umsetzbare Resultate
Bei der Entwicklung von VWO SmartStats richtete sich unser Fokus auf eine wichtige Kennzahl: Geschwindigkeit. Wir haben einen Teil der Genauigkeit für die Geschwindigkeit aufgegeben. Nicht viel, nur ein kleines bisschen, genug, um schnellere Ergebnisse zu erzielen, ohne Ihr Endergebnis zu beeinträchtigen. Dadurch gewinnen Sie Zeit und können mehr testen. Und für den Fall, dass Sie absolut sicher sein wollen, berechnen wir den maximal möglichen Verlust, den Sie in Kauf nehmen würden, und Sie können entscheiden, ob der Verlustwert Ihrer Risikobereitschaft entspricht.
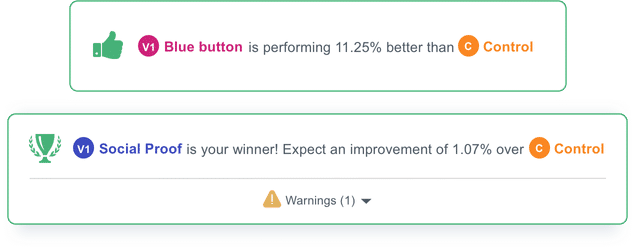
Häufig gestellte Fragen
Die Nullhypothese gibt an, dass zwischen der Kontrolle und der Variation kein Unterschied besteht. Das bedeutet im Prinzip, dass die Konversionsrate der Variation ähnlich sein wird wie die Konversionsrate der Kontrolle.
Der P-Wert ist definiert als die Wahrscheinlichkeit, ein mindestens so extremes Ergebnis wie die beobachteten zu erhalten, vorausgesetzt, die Nullhypothese ist korrekt, wobei die Variante und Kontrolle bei der Nullhypothese beim A/B-Testing gleich sind.
Die statistische Signifikanz misst, ob ein erhaltenes Ergebnis aufgrund einer Möglichkeit oder einer interessanten Tatsache wahrscheinlich ist. Wenn ein Ergebnis signifikant ist, bedeutet das im Prinzip, dass man darauf vertrauen kann, dass eine Differenz real ist (und man nicht einfach nur Glück oder Pech bei der Auswahl des Beispiels hatte).
Die statistische Trennschärfe ist die Wahrscheinlichkeit, eine Folge zu finden, wenn die Folge real ist. Eine statistische Trennschärfe von 80 % bedeutet also, dass bei 100 Tests mit unterschiedlichen Variationen 20 Tests zu dem Schluss kommen werden, dass die Variationen gleich sind und keine Folge existiert.