The Forces Behind Statistical Significance
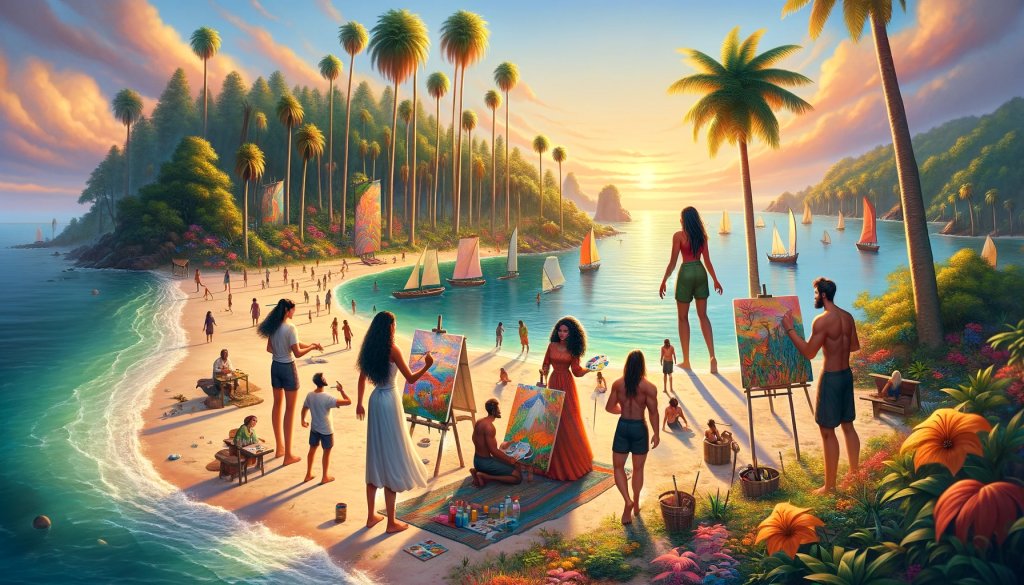
Inferential Statistics is the branch of statistics that measures if there is enough evidence to support a hypothesis or not. But contrary to classical mathematics where statements are either proved or disproved, inferential statistics does not operate in the domain of certainty. Statistics can neither prove nor disprove a hypothesis but can only indicate what is likely and not. Most inferential statistics are hence built around the idea of measuring surprise. When something happens more often than it is expected, it is surprising. When a data point is observed that is outside its usual range, it is surprising. When two things consistently behave differently from each other, for no apparent reason, it is surprising.
Let us understand this with an example. Suppose there is an island where people happen to be taller on average than anywhere else on Earth. Let us assume that globally the average height is 170 cm with a spread of 5 cm around the average. In other words, most people’s height falls between 165 cm – 175 cm.
Let’s suppose you reach the island with the assumption that people on the island are no different from the rest of the world. You then happen to see a man with a height of 175 cm. It is on the higher side but still not surprising. You probably need to see many such individuals to wonder if the evidence is surprising. However, on the contrary suppose you see just a few people with a height of 200 cm. Only a few observations with such a high deviation will be enough to convince you that the hypothesis holds. Lastly, imagine both of these cases again had the global spread of heights been much narrower, say between 169 cm – 171 cm.
Something tells us that surprise is relative and not absolute. If a metric fluctuates a lot, you are not surprised if it occasionally takes on an extreme value. However, if a metric does not fluctuate a lot, then even one large spike is enough to convince you that something has changed. Despite all the complicated stories that anyone will tell you about null hypotheses, the t-distribution, and p-values, statistical significance is nothing but a quantification of this surprise that we intuitively understand.
In this blogpost, let us explore the three forces that drive this feeling of surprise for us. In statistical terms, these are the effect size, the standard deviation, and the sample size. Together they define the formula and intuition behind statistical significance. In the next post, we will explore the relationship between these terms.
The Forces Behind Statistical Significance
You have landed on the island with tall people. You want to gather evidence for the claim that people on this island are taller than average. You start with the contradictory but simpler assumption that the people on this island are no different from the rest of the world. Statisticians call this assumption the null hypothesis. You want to figure out if the evidence is enough to surprise you against the null hypothesis.
Note that in analogy to an actual experiment, the global average of heights is like your control group (the reference point). The people on the island are like your treatment group. In the context of an A/B test, they are analogous to the existing page (control) and the new page being tested (the variant).
While you keep the analogy in mind, let us break down the factors that guide your intuition.
- Effect Size: The first thing probably that plays the biggest role is the question of how much taller people on that island are. If people on the island are giants going much beyond the average human height then you are likely to question the null hypothesis right away. However, as explained in the introduction, even if people are just noticeably taller than average people, then also seeing enough cases will convince you against the null hypothesis. In statistical terms, this is the effect size. The effect size is the difference in the mean of the control group and the treatment group. In other words, the effect size denotes how large of a metric difference you are actually observing. Note that bigger differences are much easier to spot and require fewer instances to suggest enough evidence. Smaller differences are harder to spot and require more instances to refute the null hypothesis.
- Standard Deviation: Standard Deviation is a measure of how much a metric varies by its inherent nature. We know that some metrics are much more variable than others. For instance, we know that human height usually varies only in the range of a few inches. Hence, it is surprising when we see an effect size much larger than a few inches. For comparison, imagine if the island hypothesis had not been about taller humans but taller trees. You might not have been surprised to see a bunch of very tall trees because tree heights in general have a higher variance. Unlike very tall humans, we are used to seeing very tall trees every now and then. Standard Deviation gives us an idea of the range of fluctuations that are expected in a metric. It is only when we observe an effect-size larger than the inherent variance, is when we are surprised by the evidence.
- Sample Size: The last one is probably the easiest to understand. Intuitively, we are well aware that when we see a pattern more often, we tend to additively increase our belief of the same. Similarly, when we see repeated instances of larger heights on the island, we are more and more likely to reject the null hypothesis. In that sense, even when the effect-size is negligibly small, an increasingly large sample size can build strong evidence that there is an effect. A large sample size is akin to having a high-definition picture of reality. If the picture is sharp enough, even very small differences will be clearly visible.
There is nothing more to the crucial intuition behind statistical significance. The three forces together decide whether the observed difference is statistically significant or not. If the evidence is surprising beyond a threshold, the experimenter (in our case the island visitor) rejects the null hypothesis in favor of the alternative hypothesis. He ends up accepting that there is something different with the people of the island.
You might have noticed that the island example does not exactly conform to the design of an RCT. It is hence worth noting that statistical significance is independent of the theory of RCTs. Statistical significance is just a way to measure the evidence for a proposed hypothesis. RCT is the proper experimental design that grants trust to this measure. If you calculate statistical significance in an uncontrolled environment, you can still end up with false causal insights.
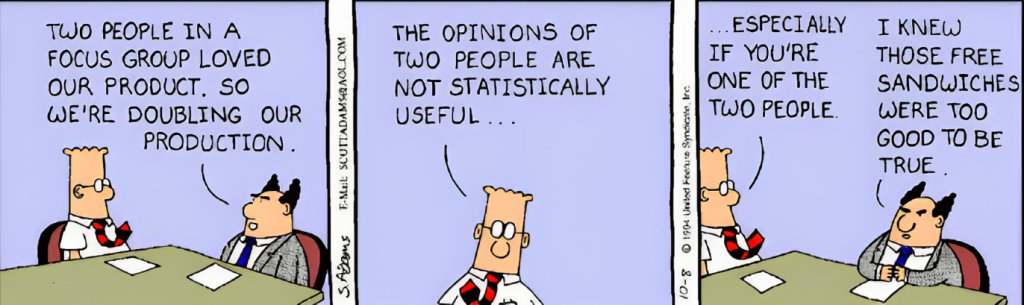
Conclusion
“Entities must not be multiplied beyond necessity”
William of Okham
This simple statement called Occam’s razor has deep philosophical implications in many domains of science. In simpler words, the statement asserts that simpler explanations are better than their more complex counterparts. From a statistical perspective, this is true because as you go on adding variables into an explanation, you go on increasing the odds of finding a false pattern purely by chance. This is what happens when we see millions of stars in the sky and try to create a constellation. As more and more stars are visible, it becomes easier to create any pattern that we can imagine.
In inferential statistics, Occam’s razor guides a deep heuristic. You do not easily give up the null hypothesis in favor of the alternative. Only when the evidence against the null becomes very strong (> 95%) is when you reject the null hypothesis. Rejecting the null hypothesis earlier (say at > 90%) might seem harmless but the Occam’s razor warns that you might start to hallucinate in increasingly complex patterns. Why the alternate hypothesis is the more complex one and not the null requires background and hence is a discussion for another day.
In the next blogpost, I will describe how these three forces come together and what theories drive the relationship between these three. The expression is crisp and easy to understand and it explains the entire crux of statistical significance.
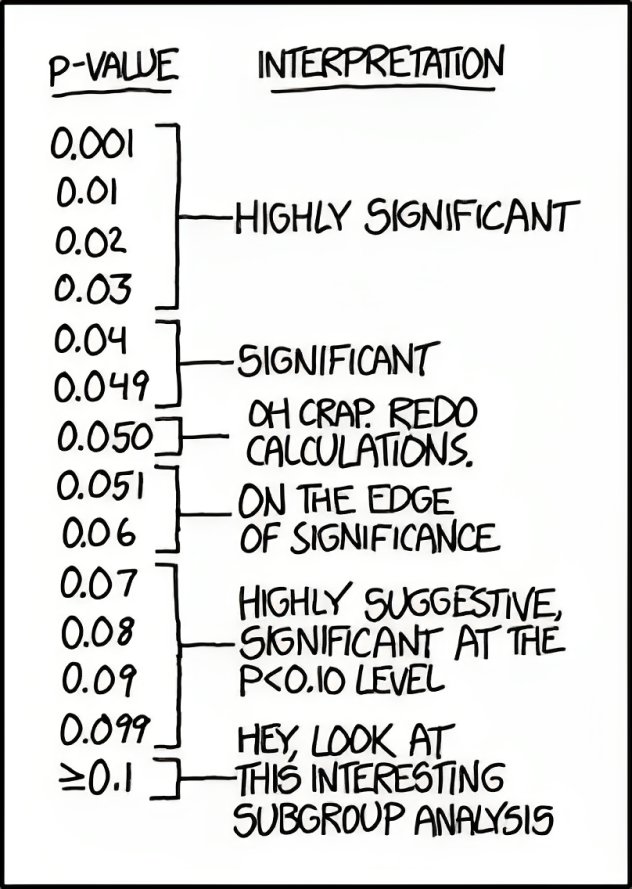