Metrics in Experimentation
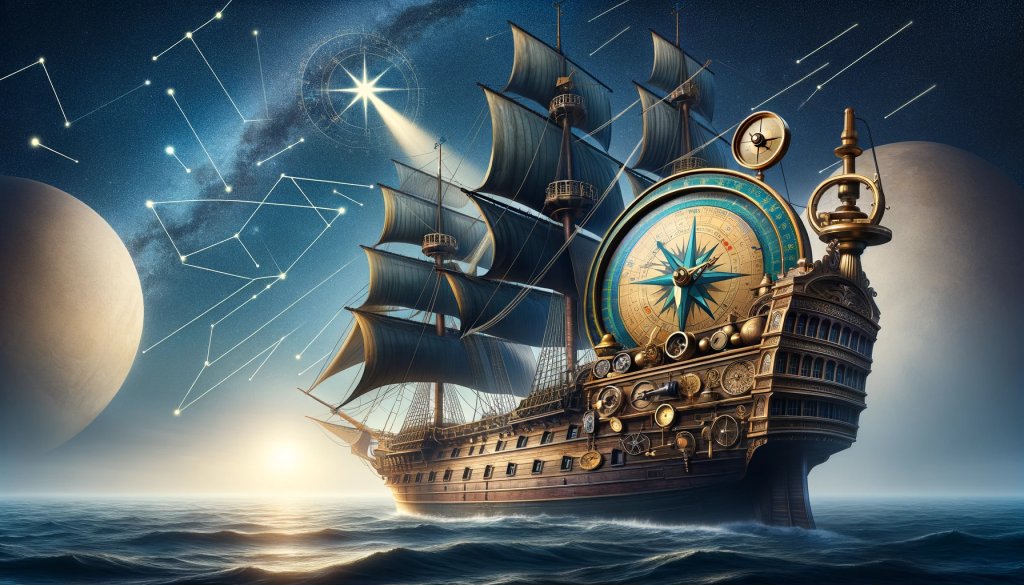
The British government in India (before 1947), concerned about the increasing number of venomous cobras in Delhi, resolved to give a monetary incentive against every dead cobra snake (the metric of choice). While the scheme worked well in the starting, some ingenious citizens started breeding cobras for the reward and started utilizing the scheme as an alternate source of income. By the time the government realized the adverse effect of the metric, the cobra population had multiplied. The scheme was scrapped, and without the incentive, the inbred cobras were let out in the open. The choice of metric had gone wrong for the British government and had exacerbated the problem rather than mitigating it.
Metrics are at the heart of experimentation because they define the difference between an idea and a hypothesis. If someone proposes an alternative way of doing things, they must also define what objective they are trying to achieve by trying this method. Only when the objective is defined does an idea become a hypothesis and can be tested via an experiment. The larger experimentation effort progresses in the direction of the metrics you choose to optimize.
But often, the direct metrics that businesses desire to optimize are lagged in time. Metrics such as quarterly revenues, customer satisfaction, and steady growth rates reflect the overall goals of a business but are too delayed for experimentation. Experimenters hence craft leading metrics that can act as a compass to lead you toward your north star. This craft of defining effective metrics to lead your ship in the right direction is deeply tricky and deeply interesting to study.
The subsection of Metrics in the VWO Stats Blog is dedicated to the study of metrics and how to choose the right metrics for your experiments. The introductory blog post gives an overview of what metrics are and the various threads of discussion around them.
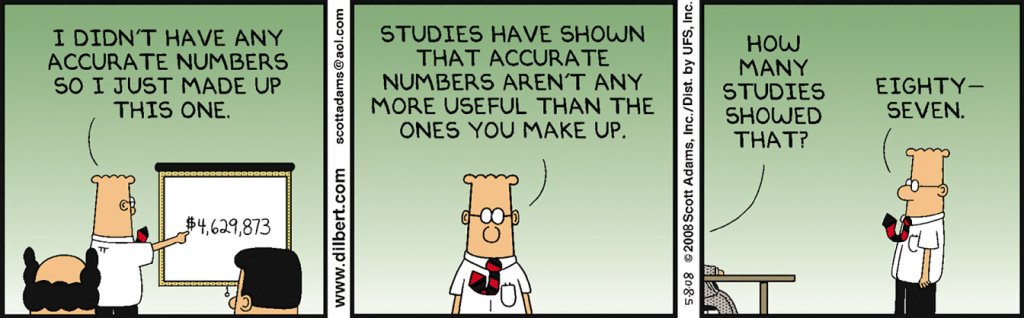
What are metrics?
We can observe and learn about our immediate surroundings through our five basic senses. But rarely have humans remained restricted to operating just in their surroundings. Our reach expands over many dimensions and much larger processes that are beyond the perception of our basic senses. A ruler who rules an entire country does not have the physical capacity to extend his vision across the entire land. A scientist who researches complex physical processes does not have the senses to measure heat, chemicals, and electricity. A CEO who leads a company obviously does not have the time to keep track of all minute processes that happen across teams. Then how as humans restricted in space and time are we able to manage systems so huge?
We simplify the multiple dimensions in a process, reduce them to tractable quantities, and then observe the entire system with these meaningful numbers. The ruler reduces the entire country to population numbers, income levels, voter demographics, and so on. The scientist looks for instruments that can track all the processes of his interest such as thermometers, chromatographs, and voltmeters. The CEO similarly reduces all processes into a hierarchy of metrics each feeding into the other and then just keeps track of the top layer metrics that he cares about. All of the above are examples of metrics that humans use to see a world much bigger than them.
Nonetheless, there are a million ways to see the same thing and they translate to a million possible metrics that you can design to track a process. But not all of these metrics correctly align with the things you care about and choosing the right ones is a mix of art and science. For instance, the total income of citizens is not a good metric to study poverty levels in a state because the more populous states will seem richer with this metric. The per-capita income is a much better metric because it takes the population into account. The study of metrics is the science of understanding how to choose good metrics that take you toward your goals and how to avoid the bad ones that hide crucial information from your vision.
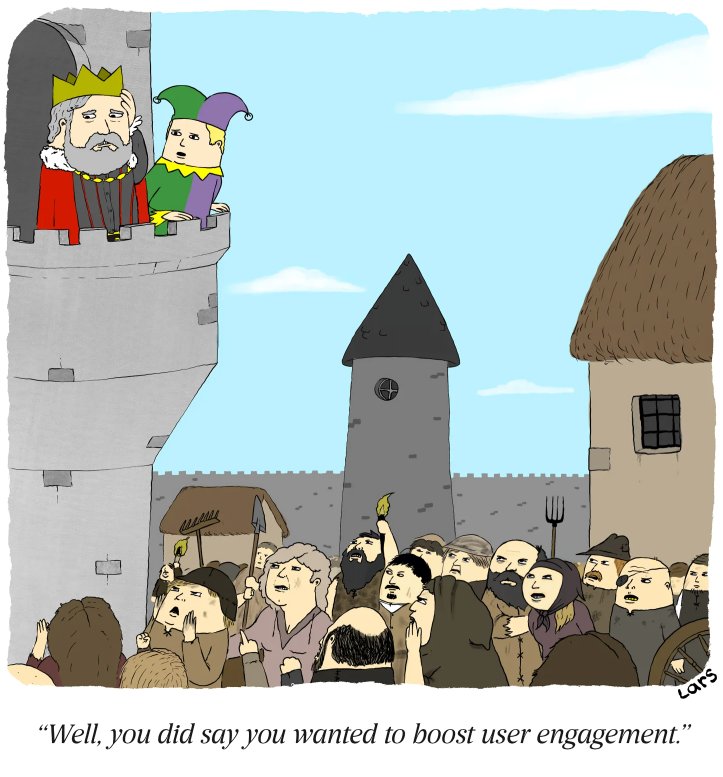
The Study of Metrics
Metrics is a topic that has been studied at length in domains beyond experimentation. Our explorations on the topic will be restricted to the aspects of A/B testing. Overall, I will take up the following threads of discussions in this subsection.
- Types of Metrics in Experimentation: Not all metrics are treated the same in experimentation and there are various categories you can divide them into. There are the success metrics that you want to improve, the guardrail metrics that you want to protect, and the diagnostic metrics that are used to better analyze the results of an experiment. The different types of metrics provide an insight into what all you can do with different metrics in an experiment.
- Characteristics of Good Metrics: Various properties are studied about metrics. Good metrics are often those that can be efficiently calculated, are sensitive to the change being made, and do not vary a lot without reason. We will study the various such characteristics that can help you craft metrics with the desired properties in mind.
- Case Studies on Metrics: The most interesting learnings around metrics come from actual stories of the struggles experimenters have gone through in designing good metrics. Case studies on metrics highlight the common mistakes experimenters make and why in the moment the metric of choice seemed obvious and correct.
- Tips, Tricks, and Fallacies in Designing Metrics: Many scientists and experimenters have devised universal laws and concepts around metrics that can help build a perspective on metrics. Badly designed metrics have been notorious throughout history for causing unexpected problems and losses to their designers. Knowing a library of tips, tricks, and fallacies in metric creation will help you build the intuition for foreseeing potential errors in metric design and correct them in advance.s
- Evolution of Metrics: Lastly, we will explore the evolution of metrics in modern tech companies and the process under which good metrics are developed and refined that eventually go on to lead the system. You usually start by tracking simpler signals and progressively improve the overall stack of tracked metrics. Eventually, as a trustworthy system of metrics is built, it demands lesser work and allows you to liberate experimentation across your organization.
Metrics is not a new concept in history but it has evolved dramatically with the explosion of data. Understanding metrics in the 21st century is the key to driving your business towards desired goals.
Conclusion
The eye of an organism has such a complex structure that many scientists have argued that it proves the existence of a creator. They say that the structure of an eye could simply not have been created in a process of evolution.
However, evolutionary theorists tell us that the eye developed under a very hyper-aggressive process of natural selection. The first form of eyes must have existed because a random DNA mutation led to a cell being photo-sensitive for the first time. It probably allowed the host organism to only differentiate between day and night. However, even a slight capability of vision gave the organism a drastic edge over other organisms, and within a few generations, any organisms without eyes were wiped out. From there on, small mutations that lead to improvement in vision gave an aggressive edge of survival to its hosts. Hence, eyes evolved at a much faster rate than any other organ.
Today, good metrics provide the same competitive edge to us that eyes provided to the initial species. History has shown us that those with a better vision are bound to dominate the pool.
The study of metrics is hence, indispensable.
(Some portions of this blogpost were written in collaboration with Manisha Arora. A special thanks to her for the same.)
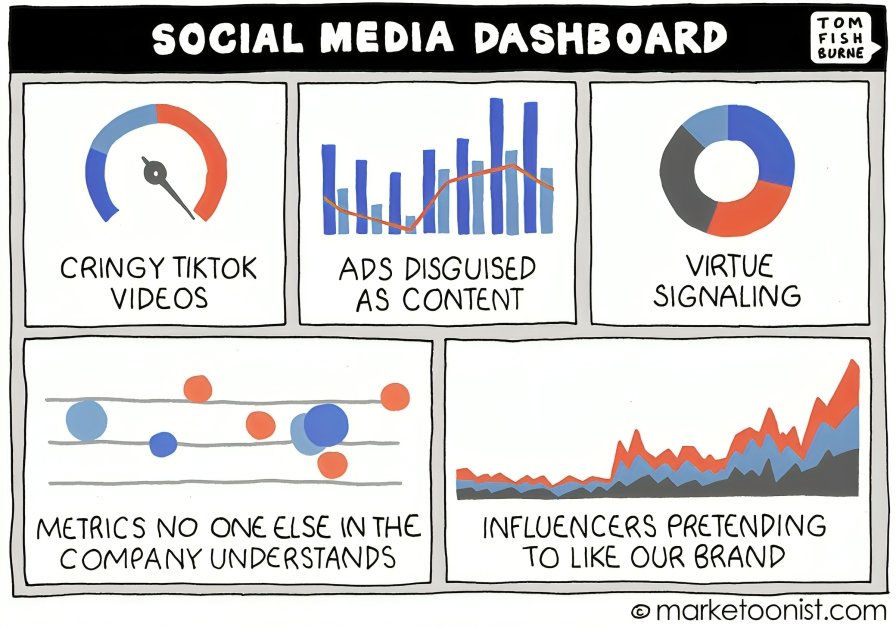