Limitations of Randomized Controlled Trials
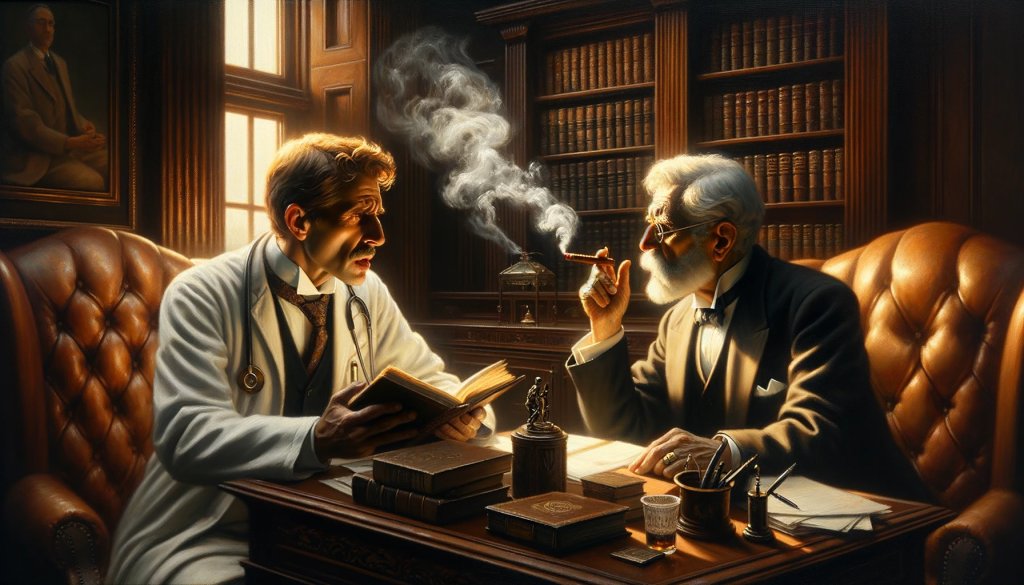
Randomized Controlled Trials solved a crucial problem for scientists in theory and they promised the world a gold standard for causal estimation. However, in practice, not all causal relationships could be estimated and RCTs came with their own set of limitations.
One such limitation came into play when the mid-20th century witnessed one of the most high-profile medical debates of the century: Does smoking cause cancer or not?
Statisticians and medical researchers clashed over the association as unavoidable evidence was collected that smoking and cancer are correlated. However, statisticians struck back with the claim that correlations are not causation. They said there may be a smoking gene that is responsible for both cancer and the craving for tobacco (a confounder basically).
If that is the case, the correlation between smoking and cancer would not be causal, and quitting smoking would not impact the gene (which will still cause cancer).
The debate was unusually personal for many, as many scientists and statisticians were themselves passionate smokers.
RA Fisher, the father of modern statistics was himself a smoker and a defendant of tobacco. He knew that a randomized controlled experiment (which he had invented) would put the debate to bed. But an RCT to establish causality between smoking and lung cancer was both difficult and unethical. Even if someone were to sign up for such a study, forcing the treatment group to smoke for an extended period had irreversible health consequences.
In the lack of a controlled experiment, the debate took interesting turns between scientists, doctors, capitalists, and policymakers for almost 30 years before getting settled by the end of the century.
This blog post introduces the reader to some of the fundamental limitations of Randomized Controlled Trials that play a role in modern-day A/B testing.
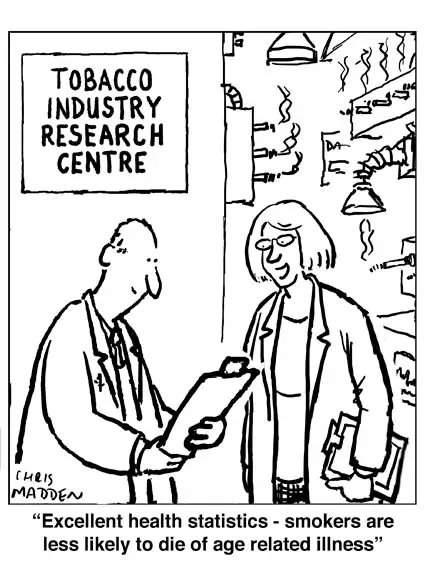
Interventions are Often a Luxury
There are two distinct ways in which you can study patterns in data. The first is the observational approach where you just collect data and observe the patterns in the data. The second is the interventional approach, where you intervene and explicitly cause something to change in the dataset (the treatment). While the difference is nuanced, it matters the world to statisticians and it is not a valid RCT until the coin toss decides who gets the intervention and not.
To understand this further, observe that there is a difference between finding a group of people who smoke by their own choice, and deciding by coin toss who in your study will be smoking for the rest of their lives and who will not. While on the outside, the two datasets might look the same, there is a world of difference that matters to statisticians.
The group that smokes by their own choice might be made up of all the people with the “smoking gene” which also causes them to die early. However, in the latter group the people with the “smoking gene” get equally distributed in the control group and the treatment group.
The most persisting limitation to the design of randomized controlled trials is that it still requires you to intervene explicitly. Making an intervention is often difficult, unethical, or costly. Some examples of this in modern A/B Testing can be as follows:
- A difficult intervention to make: Suppose someone wants to test if giving a subscribe panel at the end of the home page, makes people subscribe more to the website. However, what if most customers assigned to the treatment group do not scroll down to the bottom of the page and hence don’t see the panel at all.
- An unethical intervention to make: Suppose a social media website wants to test if showing politically polarized content impacts the kind of ads people click on. While the experimental design does not have a problem, it is highly unethical to make such an intervention to a specific group of people.
- A costly intervention to make: Suppose someone wants to test the impact of giving free coupons on the engagement of customers. However, the intervention is costly and can only be given to a small set of people which may not be enough to run a sufficiently powerful experiment.
Sampling Biases
Classically in Randomized Control Trials, often it is a huge problem to be able to collect a sample that properly represents the population your hypothesis is concerned about. The problem with such studies was that the results of the experiment often came out to be invalid when they were assumed for the larger population. For instance, a psychological study conducted in north India might not be valid in a state of South India because beliefs and societal conditioning are different.
In the context of modern A/B testing, most of these problems have been mitigated as online experimenters have the luxury to directly test on a randomized proportion of the actual population of interest. However, sampling biases still occur in some forms in modern A/B tests. Some examples of these sampling biases are as follows:
- A bias on the axis of time: Suppose you run an experiment that overlaps with the Black Friday sale due to which results get biased and are not applicable in off season.
- An imbalance in visitor properties: Suppose your A/B test happens to crash more often for iPhone users than it does for Android users and hence your sample under-represents the financially richer class. This can cause the results of your study to not be applicable to the overall visitor group.
- An imbalance between variation and control: Suppose your variation takes a longer time to load due to a redirect in the A/B test and hence it ends up crashing more often than the control. It might be that you see a lower conversion rate on variation than on control, only because your variation crashed more often.
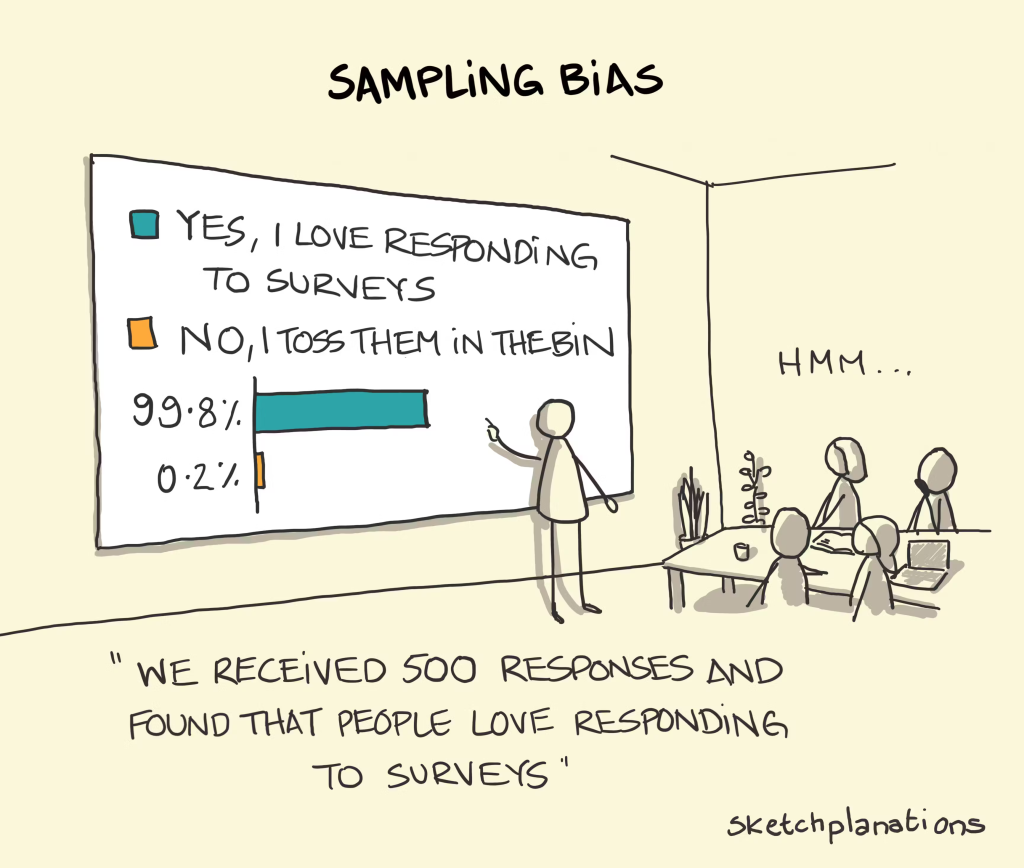
True Randomness vs Pseudo-Randomness
The crux of Fisher’s invention is the randomization procedure that decides which subject gets the treatment. This required all scientists to devise ways to generate a sequence of randomly generated numbers. However, when computer scientists started to deal with the problem of generating random numbers via algorithms, they realized that it was impossible to generate a sequence of numbers that was truly random.
Today, all random number generating algorithms require a seed which is what grants the perception of randomness to the sequence of numbers. If the same seed is used, the exact same sequence of numbers can be recovered. For the modern-day experimenter, this is not a frequent problem but as a practice, it needs to be taken care that the seed is in no way associated with the treatment being given.
Conclusion
As you read further into experimentation and statistics, you will realize that a lot of what is guaranteed in statistics is based on the idea of true randomization and a sufficiently large sample size. In reality though, both assumptions end up being idealistic in many real-world scenarios. For such scenarios where an RCT cannot be run, various alternate algorithms and tools have been proposed. Some examples of these methods are the Interrupted Time Series, the Regression Discontinuity Design, and Instrumental Variables. These methods have been grouped under the broader category of Quasi-Experimental Designs.
But these come with their assumptions and constraints that are often more challenging to meet. Despite all their limitations, RCTs provide one of the sharpest ways to cancel out all confounding biases in a causal study and isolate the change being studied.
For the same reason, A/B tests continue to be the gold standard of causal discovery available to modern tech-experimenters.